Integrating Conversational Agents with Text Clustering: Developments and Practical Uses
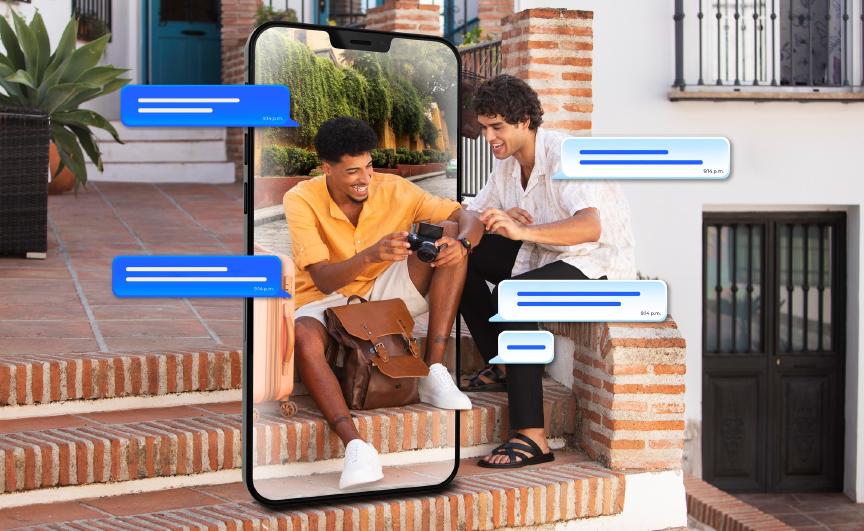
The union of chatbots and text clustering technology is shaping the future of business data management. Together, they're redefining how companies handle vast textual data, leading to enhanced user engagement and operational innovation.
This article explores the merger of these advanced technologies, highlighting their significant effects across diverse industries. Dive deep with us into this alliance's practical implications and breakthroughs, showcasing how businesses can leverage it to remain at the cutting edge of user-focused innovation.
What are Conversational Agents?
Conversational agents, commonly referred to as chatbots, represent a forefront innovation in artificial intelligence. These digital entities are meticulously crafted to emulate human-like conversations, reshaping contemporary communication. They are engineered to understand, process, and respond to human language, fundamentally altering how businesses and organizations engage with their audiences.
The significance of chatbots lies in their ability to deliver instantaneous and responsive communication. They are invaluable tools in modern communication by providing round-the-clock support, guiding users, and responding to inquiries. Their versatility empowers organizations to engage with their audience personally, making interactions more dynamic and user-centric. As they evolve, a chatbot will embody the future of human-machine communication.
Evolution and Importance of Chatbots and Conversational Agents
The journey of conversational agents, from basic rule-based chatbots to advanced conversational AI-driven entities, represents a remarkable technological evolution. Initially reliant on predefined rules and keyword recognition, these agents have evolved into advanced systems thanks to natural language processing (NLP) advancements and machine learning.
The importance of conversational agents cannot be overstated in today's digital landscape. They have transitioned from rule-based tools to sophisticated entities capable of understanding context, predicting user behavior, and delivering tailored responses. This evolution enhances customer engagement, streamlines processes, and drives efficiency across diverse sectors.
In summary, the evolution of these dialogue systems underscores their growing significance. From humble beginnings to sophisticated AI-driven solutions, they shape interactions and business operations.
Real-world Applications
Conversational agents have transcended innovation to become indispensable in real-world applications. Their adaptability allows them to address diverse scenarios and challenges across industries.
In customer service, these agents offer real-time assistance, enhancing shopping experiences on e-commerce platforms and providing essential support in healthcare for preliminary diagnosis and medical advice.
Beyond these domains, conversational agents serve as intelligent tutoring systems, financial advisors, and more, highlighting their wide-reaching impact and potential for innovation across diverse industries.
Delving Into Text Clustering
Text clustering is the art and science of grouping textual data based on similarity, akin to organising a vast library into specific genres for easy navigation. It simplifies data management and retrieval, offering invaluable utility across domains.
Text clustering's significance lies in transforming extensive textual data into structured categories, streamlining search processes, and providing valuable insights. This process underpins information retrieval systems, recommendation engines, and content organisation strategies, enhancing user experiences.
The Role of Natural Language Processing (NLP)
At the heart of text clustering lies the pivotal role of Natural Language Processing (NLP). NLP is the cornerstone technology enabling machines to comprehend, interpret, and even generate human language, ushering in a new era of efficiency in text clustering. It equips machines to navigate the complexities of language, making sense of nuances, semantics, and context, which is vital for effective clustering.
NLP empowers text clustering algorithms to go beyond mere keyword matching and delve into the deeper layers of textual content. It allows machines to grasp the meaning of words and phrases, identify synonyms and related terms, and discern the context in which they are used. This linguistic intelligence is indispensable in ensuring accurate and context-aware clustering, enhancing the precision of grouping similar texts.
By harnessing NLP techniques, text clustering becomes more than just a categorization exercise; it evolves into a sophisticated knowledge discovery process. It enables the extraction of valuable insights from textual data, helping businesses uncover trends, understand customer sentiment, and improve content recommendations. As NLP continues to advance, its integration with text clustering promises even greater accuracy and sophistication in managing and extracting knowledge from textual information.
The Integration Process
Integrating conversational agents with text clustering represents a compelling synergy between two cutting-edge technologies. This intricate process involves merging the capabilities of conversational agents, which excel in understanding and generating human language, with the precision of text clustering, which efficiently organizes vast textual datasets based on similarity.
Benefits of Merging the Two
When conversational agents and text clustering converge, the outcome is a transformative fusion of AI capabilities that amplifies the user experience. This integration is a gateway to personalized, efficient, and inherently user-centric interactions. By merging the conversational prowess of AI-driven agents with the precision of text clustering, users can expect tailored responses and recommendations that cater to their needs and preferences. This synergy ensures that users receive not just information but the most relevant and context-aware insights during every interaction, making their engagement more meaningful and satisfying.
Furthermore, integrating conversational agents with text clustering offers a dynamic way to sift through vast repositories of textual data. It empowers these virtual agents to understand the content and identify patterns, trends, and nuances that might elude manual sorting. Users benefit from the efficiency of this combined approach, as it streamlines the process of accessing information and discovering relevant content. Whether in customer support, content recommendation, or information retrieval, the amalgamation of conversational agents and text clustering brings forth a powerful alliance that enhances the utility and impact of AI-driven systems.
Steps to Successful Integration
Integrating conversational agents with text clustering is a multi-faceted process that requires careful planning and execution. To ensure a seamless and effective integration, consider the following steps:
Understand the Specific Needs of Your Audience
Before embarking on the integration journey, deeply understanding your audience's requirements and preferences is crucial. Conduct thorough research to identify the types of conversations and information your users seek. Are they looking for product recommendations, customer support, or information retrieval? Understanding these specifics will help you tailor the integration to precisely meet your users' needs.
Additionally, consider the nuances of your audience, such as language preferences, cultural sensitivities, and communication styles. These insights will guide the development of conversational agents that resonate with your target demographic, ensuring a more personalized and practical user experience.
Choose the Right Conversational Agent Platform
Selecting the appropriate conversational agent platform is a critical decision in the integration process. Evaluate various platforms based on their capabilities, scalability, and compatibility with your existing systems. Look for platforms that offer robust natural language processing (NLP) powers, which will be pivotal in enabling effective communication and understanding between the agents and users.
Consider factors such as deployment options (cloud-based or on-premises), integration APIs, and the availability of pre-trained language models. Additionally, assess the platform's support for multilingual interactions if your user base spans different languages and regions. Choosing the right platform sets the foundation for a successful integration.
Implement Advanced NLP Techniques for Efficient Text Clustering
The heart of successful integration lies in extracting meaningful insights from textual data. Implement advanced NLP techniques to better the efficiency of text clustering. It can include employing algorithms for natural language understanding (NLU) to grasp the context, intent, and sentiment within user queries.
NLP techniques can also help identify and extract critical entities, topics, and themes from the text, facilitating more accurate clustering. Explore deep learning models like recurrent neural networks (RNNs) and transformer models like BERT to empower your system to handle complex language structures and nuances. Additionally, consider using topic modeling algorithms like Latent Dirichlet Allocation (LDA) to group related content effectively.
Continuously Train and Refine the System Based on User Interactions
Integration is an ongoing process that requires continuous improvement. Collect user feedback and interaction data to fine-tune the performance of your conversational agents and text clustering algorithms. This iterative approach ensures the system becomes increasingly adept at understanding user needs and delivering relevant content.
Regularly retrain the NLP models with new data to keep them up-to-date and aligned with changing user behaviors. Implement A/B testing to evaluate different clustering strategies and conversation flows and make data-driven decisions. By actively monitoring and refining the system, you can adapt to evolving user demands and deliver a seamless and satisfying experience over time.
Incorporating these steps into your integration process will help you successfully merge conversational agents and text clustering, resulting in a robust and user-centric AI-driven solution.
A Scoping Review of the Practical Applications of this Integration
Integrating conversational agents with text clustering technology opens doors to many practical applications across diverse domains. Below, we explore these applications in various sectors, revealing this integration's versatility and transformative potential.
Healthcare
Picture a scenario where a patient engages with a conversational agent for medical guidance. The system employs natural language processing (NLP) techniques to comprehend the patient's symptoms and concerns. It then uses advanced deep learning algorithms to cluster relevant medical information, extracting insights from a vast database of health data. This integration can lead to remarkable applications in healthcare.
In addition to symptom-based diagnosis and guidance, conversational agents combined with text clustering can be utilised in areas such as mental health support. The system can assess emotional well-being by evaluating patient responses using sentiment analysis and offering appropriate interventions or referrals. Furthermore, this technology can be extended to conduct systematic reviews of medical literature, aiding researchers and healthcare professionals to stay updated with the latest advancements and treatment options. The possibilities within healthcare are vast, with applications ranging from patient engagement to medical research and beyond.
E-commerce
For e-commerce platforms, integrating conversational agents with text clustering translates into an enriched customer shopping experience. As users navigate online stores, conversational agents can analyze their interactions, queries, and preferences in real-time. By leveraging text clustering, the system can categorize products effectively, making personalized product recommendations based on user behavior and past purchases.
Additionally, this technology can power review and opinion mining, allowing e-commerce platforms to extract valuable insights from customer reviews and feedback. Text clustering can group similar reviews, summarize sentiments, and highlight key product attributes, offering a comprehensive understanding of customer opinions. This data-driven approach enables retailers to refine product offerings, improve customer service, and optimize marketing strategies. E-commerce businesses can harness this integration to boost sales, build stronger customer relationships, and enhance user satisfaction.
Customer Support
In the realm of customer support, the integration of conversational agents with text clustering streamlines query resolution processes. When users seek assistance, the system can swiftly analyze their inquiries, extract relevant keywords, and utilize text clustering techniques to group similar queries into categories. This clustering allows for efficiently presenting predefined solutions or routing more complex issues to human agents.
Moreover, sentiment analysis may be used to gauge user satisfaction and emotions during interactions. The system can tailor responses to address user concerns and enhance the overall support experience by understanding user sentiments. This integration is not limited to traditional customer support but extends to fields such as IT helpdesk services, where it can expedite troubleshooting and issue resolution.
Challenges and How To Overcome Them
Obstacles concerning conversational agents and text clustering.
The fusion of conversational agents and text clustering technology presents a transformative opportunity but also introduces a set of challenges that must be proactively addressed. Ignoring these challenges can hinder the seamless functioning of the integrated system and result in subpar user experiences. Therefore, a forward-thinking approach is essential to ensure a successful integration.
The significance of data privacy and data protection adherence.
In the context of healthcare applications, where patient data is concerned, data privacy is paramount. Conversational agents may interact with users who share sensitive medical information, making it imperative to comply with stringent data protection regulations like GDPR (General Data Protection Regulation) in Europe or HIPAA (Health Insurance Portability and Accountability Act) in the United States. Adherence to these regulations involves robust encryption techniques to secure data in transit and at rest, anonymization of user data to remove personal identifiers, and secure storage practices to prevent unauthorized access. Failure to address data privacy concerns can have legal and ethical repercussions, making it a top priority in the integration process.
Interactional quality control can be easily overlooked.
One of the fundamental objectives of integrating conversational agents with text clustering is to provide users with accurate and relevant responses. Poorly executed interactions can lead to user frustration and erode trust in the system. To ensure the quality of interactions, a continuous refinement process is essential. Such a process involves fine-tuning the language models used by conversational agents, ensuring they understand user queries correctly and provide contextually relevant responses. User feedback mechanisms are integral to this process, offering insights into areas where improvements are needed. Continuous monitoring and evaluation of interactions help identify inaccuracies or misunderstandings, enabling iterative improvements to enhance user satisfaction.
Utilizing NLP techniques and language models for multilingual interactions.
In a globalized world, multilingual interactions are increasingly common. Conversational agents must accurately comprehend and respond to different languages and dialects to cater to diverse user bases. This challenge calls for integrating advanced Natural Language Processing (NLP) techniques capable of handling linguistic nuances, regional variations, and language-specific contexts. Maintaining language models for each supported language is essential to ensure a consistent and high-quality user experience. These language models should be regularly updated to reflect language evolution and user preferences, underscoring the dynamic nature of multilingual interactions.
Issues relating to scaling in high-volume applications.
Scalability is critical, particularly in applications with a substantial user base. As users and interactions grow, the system must efficiently handle increased loads while maintaining response times and interaction quality. Achieving this requires meticulous infrastructure planning, including cloud-based solutions that scale dynamically to meet demand. Load balancing mechanisms optimize user requests across server resources to prevent bottlenecks and ensure a smooth user experience, even during peak usage. The architecture should also incorporate distributed computing approaches to process and respond to a large volume of interactions efficiently.
Universal integration challenges are solvable with universal approaches.
Continuous learning, feedback mechanisms, and data analysis are essential to overcoming common integration challenges. Addressing the challenges of integrating conversational agents with text clustering is an ongoing process that demands continuous learning and improvement.
Regular feedback loops involving user testing and analysis of interaction data are vital to refining the integration. This iterative approach allows organizations to identify areas of improvement, rectify issues, and enhance the overall user experience. It also ensures that the integration remains adaptable and aligned with evolving user needs and technological advancements, ultimately realizing the full potential of this powerful fusion of technologies.
Final Words
In conclusion, the fusion of conversational agents with text clustering is a transformative force in today's technological landscape. It has transcended novelty to become a catalyst for reshaping industries across the board, promising enhanced user experiences and tangible operational efficiencies.
As we journey forward in the era of AI integration, it becomes increasingly evident that businesses and organizations that embrace this technology will not only meet but surpass the expectations of their users. With the competitive edge it provides, they are poised to thrive in a world where seamless, intelligent, and user-centric interactions are the cornerstone of success.
Get a glimpse into the future of business communication with digital natives.
Get the FREE report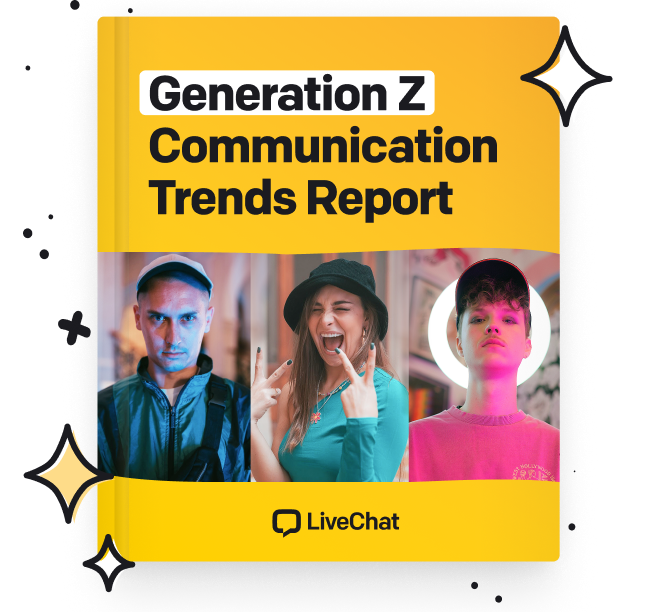