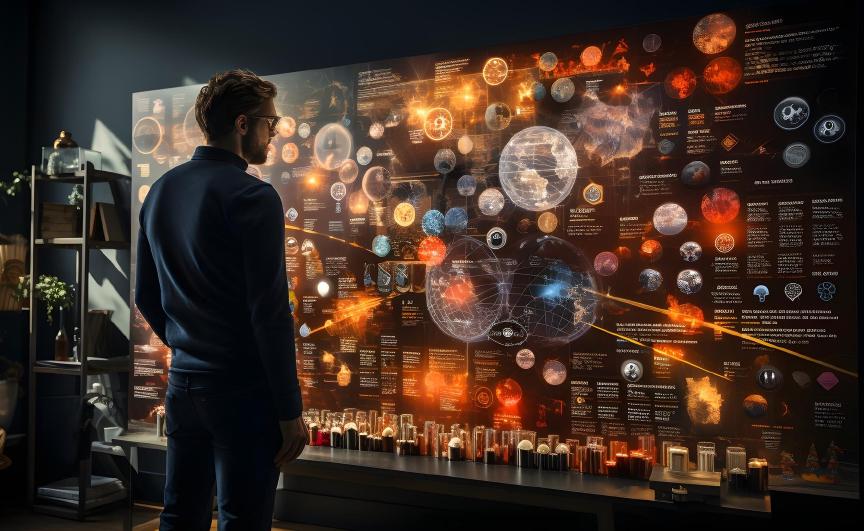
In customer service, data analysis involves gathering and evaluating data from several touchpoints, such as client feedback, website comments, call center interactions, and in-person interactions.
One of the best ways to understand existing customers, inspire their loyalty, and attract new clients is by establishing a customer-centric organizational structure and culture. By prioritizing customer experience (CX) at all touchpoints, you can generate critical insights from CX analytics that are quantifiable, actionable, and profitable.
The most common key performance initiatives (KPIs) which CX analytics draw data from are as follows:
-
Customer satisfaction: Measuring customer satisfaction helps businesses build a solid brand image, inspire customer loyalty, and lower churn
-
Net promoter score (NPS): Your NPS measures client brand loyalty and tells you whether clients will refer you to others
-
Customer effort score (CES): CES measures how easily customers use your goods or services.
Shift towards narrative-driven customer communication
Gone are the days of traditional data analysis, during which dealing with data was left exclusively to the data and analytics teams. Modern organizations are now adopting open access to data to integrate valuable data analysis techniques and insights for better business performance.
This also signals a shift toward narrative-driven customer communication based on data and utilizing data storytelling to create clear, concise, and actionable narratives around data.
What is a data story?
A data story is a data-based narrative from datasets that contextualizes raw data into broader implications specific to an organization. Whereas data science and business intelligence are focused on the technicals of translating data into insights, data stories take it one step further by solidifying these insights with domain expertise and qualitative analysis.
The overarching goal is to understand business goals and objectives better.
What is data storytelling?
Data storytelling entails crafting a narrative through data, contextualizing it accordingly, and presenting it to an audience. Data storytelling employs various data analysis techniques, including data analytics, statistics, data visualization, qualitative analysis, and contextual analysis.
Data storytelling is crucial in the modern digital world as it allows the narrator or storyteller to contextualize data into broader business objectives and use visual aids to make results more understandable. As such, audiences can easily grasp data and understand its implications regardless of technical background, domain expertise, and field of specialization.
Data storytelling takes old-school analytics further by allowing people with varying learning styles to find ways to better understand the information at hand. For example, a narrator can use various communication methods to make the data more accessible to different audiences. Auditory learners may connect well with a spoken presentation, whereas visual learners may pick up and absorb the most from data visualizations and similar visual aids. Overall, though, an excellent and well-rounded data story includes a combination of various components that can engage diverse audiences.
The concept of storytelling in brands isn’t new. More commonly in marketing, brands like Disney have long used storytelling to evoke a feeling of nostalgia and wonder to promote their products to audiences.
In Disneyland Paris’s “The Little Duck” advert, for example, storytelling is used on many levels to showcase the brand’s animation expertise and ground the message on the classic appeal of Donald Duck.
-embed if possible: https://www.youtube.com/watch?v=G4qMqbL9ACo-
Data storytelling employs the same techniques of interweaving messages to appeal to audiences but with different objectives than marketing storytelling.
The role of automation in data storytelling
Automated text communication is pivotal in transforming raw data insights into meaningful and personalized customer interactions. In today's data-rich landscape, most businesses have more data than they know what to do with.
With this level of raw data, automation is crucial to allow people to access information in manageable, organized chunks to understand customer preferences and behavior. Automated communication tools are indispensable mediators, converting these insights into targeted and relevant messages.
Through sophisticated algorithms, automated tools enable companies to craft timely and personalized content, enhancing customer engagement and satisfaction. Whether through chatbots, automated emails, or messaging platforms, these tools streamline communication processes, ensuring efficient and consistent interactions.
By leveraging the power of automation, businesses can respond promptly to customer inquiries and proactively anticipate needs. This fosters a seamless and dynamic customer experience. The integration of automated text communication not only optimizes operational efficiency but also cultivates stronger and more meaningful connections between businesses and their customers.
Tips for transforming raw data into customer-centric stories
Crafting a compelling data narrative uses elements similar to classic storytelling: Characters, setting, conflict, and resolution. Here are some tips for creating an effective data story:
Uncover what your data is saying
Before you can tell an effective story, you must uncover your dataset's causal links and correlations. This will allow you to glean interesting insights that you can use for your data story — almost like a jump-off point from where you can build your narrative.
Know your audience
You’ve heard this before, but it bears repeating every time you’re trying to tell a story. You have to determine who your audience is and what works for them. In data storytelling, find out who the story will be conveyed to, their backgrounds, and what type of communication styles might work best for them.
If, for example, you’re presenting the data to non-technical groups and visual learners, like marketers and creatives—then you can adjust your presentation to make it more visual and easy to understand. If, for instance, you’re presenting to data analysts and programmers, then a different approach may be in order, as your explanations here can be more technically complex.
Filter out non-essentials
The amount of data within an organization can be overwhelming, especially when constructing a data story that has to be cohesive and understandable. As a general rule, identifying essential data can help you inform the kind of narrative you’ll be forming and the topics you’ll want to dive into.
For example, if you’re looking to tell a story about CX, relevant data will include customer feedback, and product usage and sales data. Conversely, things like employee experience may not be as relevant. Condensing your data into essentials will help you form a clear and concise data story that will not distract your audience.
Find effective visualizations
There are many types of data visualizations, so finding the most effective ones to represent your data is essential. Data visualizations are powerful ways to help your audience really grasp your data. For example, if you’re showing a trend over time, you can use a chart that exhibits time series information.
One helpful tool to help you find a visualization to represent your data best is ThoughtSpot, which uses AI to provide data visualization recommendations. Liveboards is another tool that can help you use interactive visualizations.
Contextualize
Context is crucial when creating a data story. Domain expertise is essential when forming a data narrative, as this will help you get through to your audience effectively. It’s important to weave your data insights with context effectively, and this means providing an overview, using data visualizations, and framing your data insights within the context of your organization’s reality and context.
Challenges and trends in data storytelling
Among the most common challenges in data storytelling is not delineating between exploratory and explanatory analysis, as conflating the two can result in a muddled narrative. Exploratory analysis entails understanding and exploring data without holding onto any specific hypotheses, biases, or preconceived notions before formulating your story.
On the other hand, explanatory analysis focuses on explaining something right off the bat using the data you have. So, you should be clear on what type of analysis you are going for before you begin your data narrative.
Not understanding your data is another common challenge when building a data story, as this may result in a false interpretation or poor contextualization of your data insights. Additionally, choosing the wrong data visualizations may hinder the effectiveness of your storytelling, as you may mislead or confuse your audience.
As for emerging trends, wider data literacy is at the top of the pile as more organizations are better able to understand just how valuable data is. A shift in organizational culture is also apparent in many companies embracing a more data-centric culture.
Additionally, many more data storytelling frameworks are coming to the forefront. These include the Situation, Task, Action, and Result (STAR) framework, the And, But, and Therefore (ABT) framework, and the What, Why, and How (WWH) framework.
Conclusion
Formulating a compelling data narrative involves effective data analysis and visualization. But before that, it must be hinged on a solid understanding of what is essential from your wealth of data. Using a storytelling approach also means that you’re considering your audience, how they learn, and how they communicate so you can find the best ways to help them understand what you’re presenting.
Get a glimpse into the future of business communication with digital natives.
Get the FREE report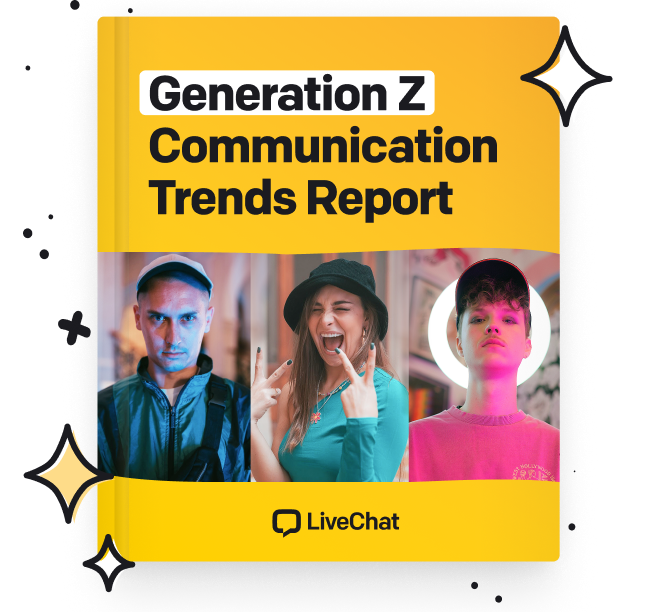