The Impact of NLP, Text Analytics, and Language Detection: Transforming Customer Interactions
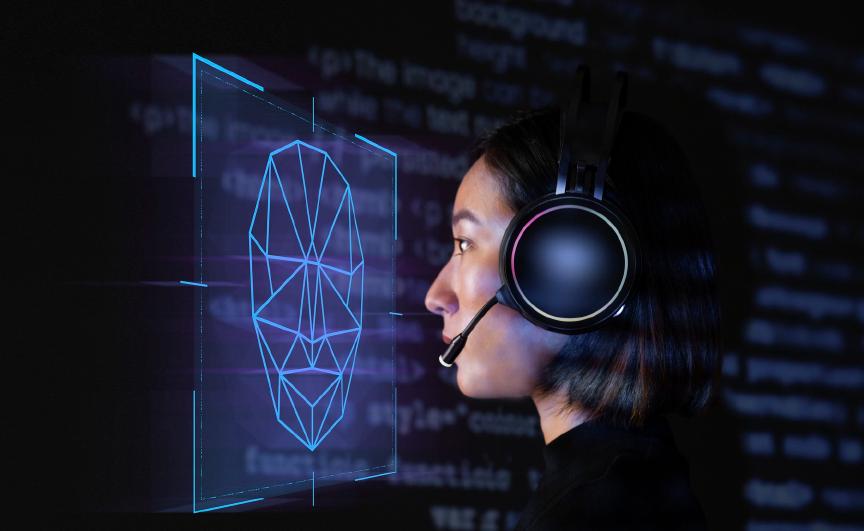
Customers are constantly communicating. Whether it's a 5-star review about a recently purchased product or the harvested text from a customer support call, there is now a sea of data points, allowing businesses to better understand their customer base.
But how exactly are organizations using this data to enrich their customer interactions?
This article explores the “how” behind this vital question. It shows how the blend of natural language processing (NLP), text analytics, and language detection has transformed the way businesses interact with their past, current, and prospective customers.
Understanding NLP and its role in customer interactions
Natural Language Processing within customer service
Natural language processing is the broad application of computer science, artificial intelligence (AI), and linguistics, focusing on the interaction between humans and computers using natural language.
Due to this natural ability to decipher the meaning and intent behind spoken and written language, NLP has become a driving force in allowing customer-centric organizations to further understand human language and provide efficient, personalized, and intuitive assistance to their customer base.
How NLP comprehends and responds to human language
At its core, natural language processing strives to bridge the gap between human language and computer understanding. It applies various algorithms and techniques to analyze text or speech syntactic structure and semantic meaning.
Here are some of the most common functions that occur within a natural language processing workflow:
-
Tokenization: Breaking down text into words or phrases (tokens) to analyze them individually.
-
Text cleaning: Removing any irrelevant or unnecessary elements from the text, such as particular characters, punctuation, or stopwords.
-
Part of speech tagging: Identifying the grammatical categories of tokens (nouns, verbs, adjectives, etc.).
-
Parsing: Analyzing the grammatical structure of sentences to understand how different words relate.
-
Named entity recognition (NER): Locating and classifying elements in the text ( names, locations, dates, etc.).
-
Semantic analysis: Using word embeddings and semantic role labeling to help capture the semantic relationships between words.
-
Sentiment analysis: Detecting and categorizing opinions or emotions in the text.
These deep learning methods enable computers to understand the nuances of human language and facilitate more meaningful interactions with users.
The impact of natural language processing on automating customer interactions
The adoption of text analytics and natural language processing with language detection within customer interactions is transforming the customer service landscape in several ways:
-
Chatbots and virtual assistants: By leveraging NLP technology, chatbots can simulate human-like conversations, answering customer queries with increased precision and efficiency. By 2025, the chatbot market is forecasted to reach around $1.25B USD, making it one of the most prominent applications of NLP.
-
Intelligent support routing: NLP allows automatic routing of customer queries to the appropriate support channels or team members based on the intent and subject matter of the inquiry, increasing overall efficiency and customer satisfaction.
-
Social media monitoring: Through sentiment analysis and topic modeling, NLP enables businesses to swiftly identify trends and understand public sentiment within social media data.
Analyzing customer feedback through text analytics
Definition and significance of text analytics in customer service
Text analytics is a branch of natural language processing that extracts valuable insights from large volumes of unstructured text data.
In today's digital age, organizations can access massive amounts of unstructured data from various sources, including social media, emails, and customer reviews. Businesses can identify patterns and trends in these conversations within this data, ultimately improving customer relationships.
Mining insights from unstructured data
Unstructured data makes up around 80% to 90% of our global data, so there has to be a pragmatic approach to unraveling its complexity to pinpoint customer insights.
One of the most common approaches to organizing unstructured customer data is text mining. Text mining is turning unstructured data into structured data by combining text extraction, classification, and various statistical methods to organize and classify external data.
To get a sense of what goes into text mining, here are a few of the key concepts and the methods and techniques that commonly appear:
-
Collocation: Enhances the text's granularity and facilitates a more profound comprehension of its semantic structure, ultimately resulting in more precise outcomes in text mining.
-
Text classification: The process of assigning categories (tags) to unstructured text data, making it easier to organize and structure complex text.
-
Concordance: Used to recognize the context or instance in which a word or set of words appears.
The impact of language detection on the global customer experiences
Breaking language barriers in customer service
There are over 7000 spoken languages globally, which presents a massive opportunity for businesses to enhance all areas of their customer experiences through a multilinguistic model.
Language detection refers to discerning the language used in a given text, which is fundamental in various applications, including natural language processing, translation services, content filtering, and customer analytics.
This branch of natural language processing relies on algorithms that analyze the frequency of characters and contextual patterns within the writing, enabling services to recognize and adapt to different languages automatically.
Globally speaking, this is one of the fastest-growing areas of natural language processing. With an annual growth rate of 13.47%, the total market size is expected to expand to $4.93B USD by the end of 2023.
Enhancing customer engagement through localization
Localized content is essential for enhancing customer engagement on a global scale. When combined with cultural adaptation, machine translation in natural language processing enables the creation of personalized and contextually relevant content for customers from diverse backgrounds.
This process, known as localization, goes beyond simple language translation to consider factors such as regional dialects, idiomatic expressions, and cultural values. This approach results in more effective communication, fostering robust customer relationships and driving higher satisfaction rates.
Applications of language detection and translation
Some of the practical applications of language detection for customer experience include:
-
Chatbots & virtual assistants: Real-time translation features in chatbots and virtual assistants enable seamless cross-language communication, improving customer service accessibility and reducing response times.
-
Content adaptation: Localizing marketing and product collateral ensures the material is linguistically accurate and culturally appropriate, increasing its effectiveness and persuasiveness.
-
Social media monitoring: Automated language detection and translation tools enable businesses to monitor and analyze customer feedback in multiple languages, helping to stay tuned to the global pulse of customer sentiment.
Integration of NLP, text analytics, and language detection
Exploring the seamless integration of NLP, text analytics, and language detection
Integrating natural language processing, text analytics, and language detection offers immense benefits to businesses by improving customer interactions.
But how do these technologies get implemented within organizations?
Unfortunately, the answer is, "It depends," but it usually takes the form of web-based applications with various integration capabilities, such as REST APIs, depending on the platform.
On top of that, many platforms (depending on the amount of data) have various degrees of storage accommodations for businesses, making them highly customizable for most applications.
Below are some examples of budding NLP companies that offer this sort of integration:
-
Unstructured text data analysis: HyperGlue is a US-based startup that allows businesses to manage and analyze unstructured data sources efficiently. Due to the need for external data sources, this company offers various integrations and is entirely web-based.
-
Call center operation automation: Birch.AI is another US-based startup that uses various NLP and AI practices to automate call center activity. Their platform allows businesses to conveniently combine popular customer relationship management (CRM) and dialer systems via API connections.
-
Conversational AI: deepset is a German startup leading the way in conversation AI and question-answering technology. Similarly, their Haystack platform offers fully customizable pipelines via REST API, allowing companies to build tailored NLP solutions.
How the trifecta enhances the overall customer service experience
Integrating language technology improves the entire chain of customer service. As a customer evolves from a random lead in a CRM to a well-paying account, various changes can be addressed with these technologies.
Here are a few examples that can be used within a typical customer lifecycle:
-
Lead generation chatbots: Chatbots equipped with NLP capabilities can understand the user's intent during the conversation, allowing them to engage with potential leads in a personalized manner, asking relevant questions to qualify and understand their needs.
-
Lead nurturing with text analytics: Text analytics can analyze lead interactions with various touchpoints, such as website forms, downloads, or webinar attendance. By extracting insights from these interactions, businesses can refine lead scoring models, ensuring that the most engaged and interested leads are adequately nurtured into conversions.
-
Behavioral analysis for rewards: NLP algorithms can analyze customer interactions, feedback, and purchase history to understand individual preferences and behaviors. This information can be used to tailor personalized loyalty programs, offering rewards and incentives that resonate with each customer's unique interests.
-
Issue pattern recognition: Text analytics can identify recurring themes or patterns within customer support interactions. Businesses can use this information to proactively address common issues, reducing the number of incoming tickets and enhancing the overall customer support experience.
Challenges and ethical considerations
Addressing privacy concerns
Addressing privacy concerns is one of the main challenges in using natural language processing, text analytics, and language detection in customer interactions.
Since these technologies deal with processing and analyzing large amounts of customer data, it is essential to maintain customer data protection throughout the process. Companies need to be transparent about their data collection and usage practices and should only collect relevant and necessary data for their purposes.
There are several ethical implications related to data privacy, especially in cases where sensitive information is involved. Organizations must ensure that they comply with data protection regulations, such as the General Data Protection Regulation (GDPR) and the California Consumer Privacy Act (CCPA), and must obtain informed consent from users before collecting or processing their data. Implementing privacy-preserving techniques, such as anonymization or data encryption, can help protect customer information to a certain extent.
Maintaining data accuracy
Another challenge is maintaining the accuracy of the collected data. Like other machine learning models, natural language processing systems rely on large amounts of data to learn patterns and generate predictions.
Inaccurate or biased data can lead to incorrect predictions or actions, negatively impacting customer interactions. It is essential to ensure data quality by conducting regular data audits and processing data consistently and standardized.
By addressing these challenges and ethical considerations, businesses can enhance customer interactions while maintaining a strong focus on privacy and data protection.
Future trends and innovations
Trends in NLP, text analytics, and language detection
According to a StartUs Insights survey, the future of language technology will continue its growth into:
Virtual assistants - 18% of total industry growth.
Sentiment analysis - 18% of total industry growth.
Multilingual language models - 15% of total industry growth.
To take things even further, here are some interesting, real-world examples of companies that contribute to the growth of language technology:
-
Virtual assistance - Vox, a Malaysian startup, specializes in automating conversational experiences. Their virtual assistant interacts with customers across diverse channels and devices, accommodating various languages through conversational AI and machine learning algorithms.
-
Sentiment analysis - Y Meadows automates customer support requests through sentiment analysis. The startup's solution for customer service automation gathers customer data via various channels, including emails and web forms, with a keen understanding of human intent.
-
Multilingual language models - Lingoes, a startup based in Finland, has developed a streamlined solution for training and deploying multilingual NLP models with a single click. This platform encompasses intelligent text analytics across 109 languages, automating all the technical steps required for setting up NLP models.
Predictions for automated customer service communication
More than 50% of business leaders are increasing their investment in customer automation systems, which is a clear signal for the future of this industry.
Much of this adoption is arguably due to the growth and innovation within the NLP field, which, according to Statista, is estimated to reach $23.68B USD in market size by the end of 2023 and $63.37B USD by 2030.
Data aside, customer communication has been, and will always be, a vital component of business growth across the globe, which will continue to propel the expansion of NLP within customer-centric applications for many years to come.
Conclusion
In summary, integrating natural language processing, text analytics, and language detection presents a powerful combination for businesses seeking to elevate their customer service strategies.
Natural language processing is the fundamental technology that enables machines to comprehend and generate human-like text. Building upon NLP principles, text analytics extracts valuable insights from unstructured text data. Meanwhile, language detection is a specialized application that falls under the broader umbrella of NLP, specifically dedicated to identifying the language within a given text.
Together, these technologies offer a comprehensive suite of tools, each with its unique emphasis, collectively shaping the ever-evolving landscape of customer interactions.
Get a glimpse into the future of business communication with digital natives.
Get the FREE report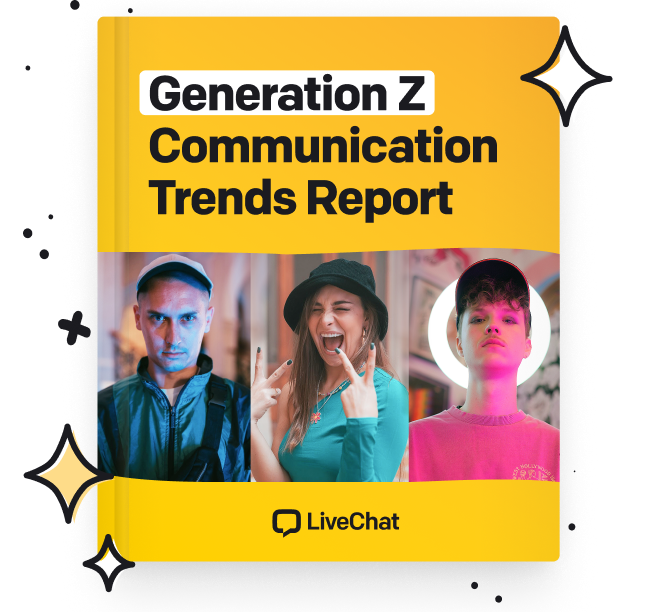