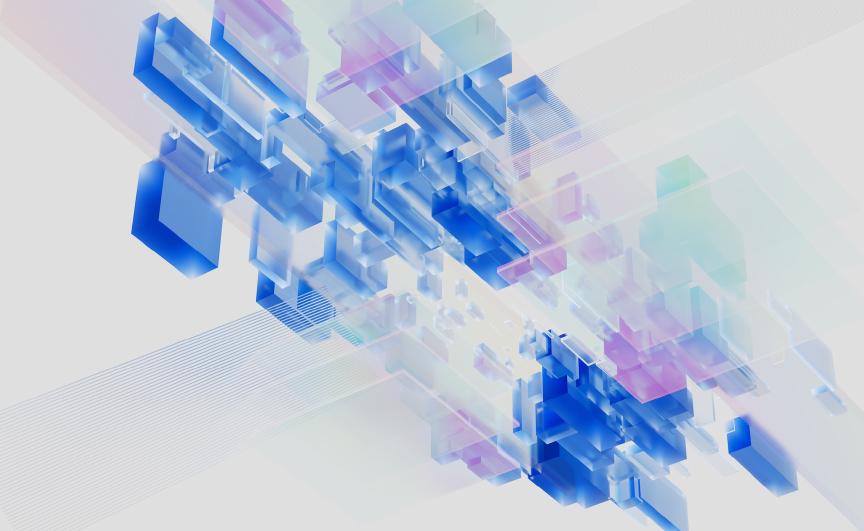
Customer experience has become somewhat of a battlefield. Data shows that 89% of businesses compete primarily based on customer experience. The modern consumer buys products that meet their needs, but the experience around the purchase, the product, and the brand are quickly becoming more important.
To gain and retain customers, brands must do more than just hone their products to perfection. They must also offer a memorable experience.
But conquering customers’ hearts requires precise knowledge. Companies must learn about customers’ needs, desires, and feelings to address them. Quantitative feedback like net promoter scores, online surveys, or ratings can give good insights into your overall performance.
However, all this research won't give you as much insight as the analysis of customer sentiment will.
What is sentiment analysis?
Sentiment analysis, also called opinion mining or emotion analysis, is a branch of computer science. It uses machine learning and natural language processing to measure the positive and negative sentiment and tone of the text, spoken language, images, and even emojis.
A sentiment analysis system is a useful tool that helps businesses to look far beyond the number of likes or shares. Through qualitative feedback, sentiment analysis helps determine whether consumer attitudes toward products, services, and brand activities are positive, negative, or neutral.
Why is sentiment analysis important in business?
Gathering customer opinions has never been easier. Live chat, chatbot conversations, ticketing systems, online surveys, social media posts, forums, and blogs are all loaded with useful customer feedback.
The problem is that being able to collect and analyze all of the user-generated content that appears online borders on the impossible. Sentiment analysis work is also very open to interpretation. Customers are humans, and we all express feelings differently.
They often apply negative and positive statements in one comment and use sarcasm.
The dinner was great, the waiter was terrible.
Great service, we were waiting over an hour for the bill
Any millennial will tell you the difficulties of understanding sentiment in text messages
To fully understand customers and their issues, you must meticulously analyze and categorize huge amounts of feedback. That’s where sentiment analysis tools come in handy. With the right training data, a good machine learning model can help automate collecting data and analyze it at scale.
Sentiment analysis tools such as Talkwaker can detect sarcasm, too. Such forms of social media monitoring helps determine what customers say, but most importantly, what they actually mean. Understanding the sentiment of the text can help fix issues and improve business processes. Therefore, accurate sentiment analysis can be used to improve the customer experience.
Types of sentiment analysis
Online businesses can use several approaches to sentiment analysis over multiple social media platforms to the opinions expressed toward their business, products, industries, or just about anything else. Training an effective sentiment analysis model will rely on several of the following models to assess textual data and provide a sentiment score:
Fine-grained sentiment analysis
Fine-grained sentiment analysis, also known as aspect-based sentiment analysis, is a model for sentiment analysis that looks beyond overall sentiment to analyze specific aspects or features of a product or service. This type of analysis can help online businesses identify which aspects of their product or service customers are most satisfied or dissatisfied with.
For example, suppose a customer leaves a review for a hotel and mentions that the rooms are spacious, but the food is mediocre. In this case, a natural language processing tool can identify these specific aspects and provide insights into what customers like and dislike about the hotel.
Emotion detection
Emotion detection is a type of sentiment analysis that goes beyond neutral, negative, or positive sentiment and instead identifies specific emotions, such as happiness, anger, or sadness. This type of analysis can be particularly useful for online businesses that want to understand the emotional reactions of their customers to their products or services.
Let's look at an example. An online retailer might use emotion detection to analyze customer comments on social media to identify not just what's being said about the product but whether their products are making customers happy or frustrated. This form of sentiment analysis can identify the emotions associated with the product and help product owners fine-tune the experience around that product.
Intent analysis
Intent analysis is a sentiment analysis model using deep learning to identify the intention behind a customer's statement. This type of analysis can be particularly useful for online businesses that want to understand the needs and preferences of their customers.
Another example: a customer leaves a review for a local pool, explaining that there were lots of modern facilities, that the staff was attentive and friendly, good value for money, but it was a little disappointing that the water slide was closed.
Automated sentiment analysis would identify this review as mostly positive since the customer used positive language to describe their experience at the pool. However, a more nuanced and sophisticated sentiment analysis that includes intent analysis would consider the reason for the negative sentiment about the closed pool.
Intent analysis would aim to understand why the customer was disappointed about the closed pool. Was it because they were specifically looking forward to swimming, or was it because they had children with them who were disappointed? Analyzing the language used in the review helps identify the intent behind the negative sentiment.
By using intent analysis as part of training a sentiment analysis model, businesses can gain a deeper understanding of their customer's needs and preferences and take more targeted and effective actions to improve customer satisfaction.
Multilingual sentiment analysis
Multilingual sentiment analysis is a type of sentiment analysis that can analyze text in multiple languages. This type of analysis can be particularly useful for online businesses operating in multiple countries or with customers who speak different languages.
Sentiment analysis can be difficult in multiple languages. Sentiment analysis uses machine learning, and what that machine learns is only as good as the data set that it processes. You can feed an algorithm the entire Lord of the Rings universe in Italian, but one can argue that such data won't help it analyze the sentiment toward buying used car parts online in and around Rome.
An e-commerce brand might use multilingual sentiment analysis to analyze the reviews of its competitor in a foreign market before deciding to expand in that region. Sentiment analysis can be applied here to help the business decide if they can do things differently in this market and, ultimately, whether it's worth expanding into this region.
Social media sentiment analysis
Social media sentiment analysis is a type of sentiment analysis that analyzes social media posts, comments and mentions to gain insights into customers' opinions and emotions. Sentiment analysis of social media can be particularly useful for online businesses with a strong social media presence and who want to monitor and engage with their customers on social media.
For example, a social media marketing agency might use social media sentiment analysis to identify customer complaints or negative comments about their client's products or services and address these issues in real-time to improve customer satisfaction.
In competitive industries, businesses can also use sentiment analysis to monitor the social media feeds of their competitors to become the more attractive opinion.
Use cases for sentiment analysis
Looking to get started with sentiment analysis? Here are several ways that your data scientists can analyze sentiment for the benefit of your business.
Brand monitoring
Social networks and the popularization of smartphones have opened a new dimension in terms of sharing and consuming data. Consumers write whatever they want online, and their voices are heard globally. That's why monitoring customer sentiment matters more than ever. Positive customer reviews build trust and boost conversion. Negative ones may ruin your reputation for months, while neutral sentiment can do more harm than good.
Data shows that 95% of customers read online reviews before making a purchase. This is why many brands send reminder emails to encourage users to leave a review and often incentivize the process.
But there’s always someone who won’t like your products or service, and they’ll be more than happy to share that online. When they do, you should know about it.
Sentiment analysis tools like Sotrender or Brand24 can help you keep your ear to the ground and perform sentiment analysis around the clock. So, if anything harmful to your brand appears online, you’ll be informed about it. With this functionality, you can handle issues immediately by countering fake news and apologizing to angry customers. By getting ahead of it, you’ll prevent unfavorable content from going viral.
Customer service
Since sentiment analysis lets you monitor the sentiment expressed in real-time and across channels, it’s a perfect tool to keep an eye on the health of your services. It’ll allow you to learn about the weak points in your customer support and help troubled customers quickly.
Businesses also apply sentiment analysis to discover what customers feel when they contact their customer support teams. This helps determine which agents succeed in delivering efficient service and which need to improve their performance to boost customer satisfaction. These insights help determine what satisfies customers and better train new customer service representatives.
On top of that, sentiment analysis helps companies that deliver support using call center software or chatbots to prioritize customer issues. Virtual agents can transfer unhappy customers to human specialists so that agents can take care of customers’ problems before they escalate. The same applies to help desk systems. Sentiment analysis algorithms can detect concern and anger in emails and mark them as cases with the highest priority. This helps customer service agents, who manage a massive number of requests, decide which issues need immediate attention.
Marketing
Sentiment analysis provides marketing intel and allows you to measure the performance of your promotional campaigns and events. Using it, you can find out whether your marketing activities generated any reactions online and then check their sentiment. This helps to rethink your strategies, if needed, and better personalize your messages in the future.
A good example of using sentiment analysis in marketing is the case of Expedia Canada, a travel company that launched its “escape winter” campaign in 2014. The campaign created quite a stir online. However, when the company took a closer look at the sentiments of the comments, it turned out that many of them were negative. The brand discovered that people hated the violin music used in their commercial. Because they were monitoring social sentiment and not just the number of mentions or shares, the brand corrected its course quickly.
Soon after that commercial aired, the brand released a spin-off video that shows a character from the first commercial breaking the violin. They hit the jackpot with that approach. The company showed that they have a sense of humor and listen to their customers, which helped to improve the social sentiment.
Sentiment analysis can help you to boost your online presence, too. If you pick up on positive comments quickly, you can immediately respond to them and fuel the discussion with customers who have just become your brand’s advocates. This also helps to build better relationships and lets you find good examples for case studies.
Product analytics
Customers’ opinions about products are influenced because of many different factors. They also change on the fly, like feature improvements, price increases, and marketing campaigns. All of these factors stir all sorts of emotions in users.
Product managers must constantly monitor how users respond to products and the changes they undergo. Sentiment analysis is useful in this area, too.
Sentiment analysis tools such as HubSpot’s Service Hub let you transform lots of qualitative data into quantitative insights on products and features. This helps to find out more about how customers perceive your solutions, how they perform on the market, and what should be done to improve their usage and reception.
Market research
Sentiment analysis isn’t the most important tool for market research. However, it can consolidate data and help overcome the deficiencies of typical research methods, such as surveys or focus groups.
By applying sentiment analysis to research techniques, brands can systematically collect data from many customers across multiple communication platforms. People share tons of experiences online every day and hour. There are more than 330 million active users on Twitter, and they send over 500 million tweets daily. They post about various things, including their experiences with brands. Analyzing and categorizing all this data lets marketers get a broader picture of the market and their competitors. This, in turn, helps to better understand customers' desires and create unique value propositions.
Stock market prediction
Stock prices are difficult to predict. They change out of the blue and depend on multiple economic and political factors. Curiously, the data shows that there is also a strong correlation between stock prices and social media sentiments. Articles, news, reviews, and even a single tweet can influence brand reputation and impact stock performance.
In 2018, when an American celebrity, Kylie Jenner, tweeted her displeasure with Snapchat, the company’s stock prices went down by 7%, losing over $1.3 billion of market value.
Source: Business Insider
The same year, one of Elon Musk’s tweets affected the stock prices of Tesla.
Source: Forbes
The relationship between social sentiment and the stock market encourages investors to use sentiment analysis and AI algorithms when considering investing decisions. By using them, sentiment analysis allows investors to discover the public viewpoint of particular brands and get hints on stock trends and movements.
Sentiment analysis challenges
Sentiment analysis is being applied to countless numbers of business cases where it has proven to be useful. Still, sentiment analysis models pose quite a challenge for researchers. The range of vocabulary we use is evolving constantly. New words appear every day, and existing ones get new meanings.
Automated and machine learning sentiment analysis can provide valuable insights for businesses, but it's not a perfect solution. Building your own sentiment analysis system can be challenging and may require expertise in natural language processing and machine learning. Additionally, the quality of the results depends on the quality and quantity of the text data used to train the model.
Sentiment classification is not always straightforward, especially when dealing with nuanced language, sarcasm, or irony. For example, a sentence like "I guess the service was okay, considering I had to wait an hour for my food" could be interpreted as positive or negative depending on the context. This makes it difficult to achieve a high level of accuracy in sentiment analysis.
Another challenge is that the application of sentiment analysis can vary depending on the industry and the specific use case. For example, sentiment analysis for product reviews might be more straightforward than sentiment analysis for customer service interactions. In some cases, it may be necessary to customize the sentiment analysis system to the business's specific needs.
One of the imperfections of sentiment analysis is that it can sometimes misclassify the sentiment of a particular text. For example, if a review says "I loved the hotel, but the room was too small," sentiment analysis might categorize it as negative because of the negative sentiment expressed about the room size, even though the sentiment about the hotel overall is positive.
Another difficulty is that understanding sentiment is not always enough. Businesses need to be able to interpret the sentiment in the context of their specific use case. A business might want to know not only whether the sentiment is positive or negative, but also what specific aspects of the product or service are driving the sentiment.
Despite these challenges, there are many applications for sentiment analysis in business, including customer service, product development, marketing, and reputation management. By analyzing customer feedback and social media posts, businesses can gain valuable insights into customer sentiment and use that information to make data-driven decisions.
Customer sentiment analysis: conclusion
Business based approaches for sentiment analysis can help companies provide a better experience for the customer. Aspect based sentiment analysis can also help businesses learn more about their ideal consumer and fine-tune their marketing message, resulting in a lower CAC and increased LTV.
While automated and machine learning sentiment analysis can provide valuable insights for businesses, there are challenges and imperfections that must be taken into account. Businesses should carefully consider their use case and the quality of their text data when building a sentiment analysis system, and be aware of the limitations and potential biases of the system. With careful consideration and interpretation, sentiment analysis can be a powerful tool for understanding and improving customer sentiment.
The long and the short of it is this: sentiment analysis helps businesses figure out what people feel toward their company, and there aren't many businesses that don't pay attention to that.