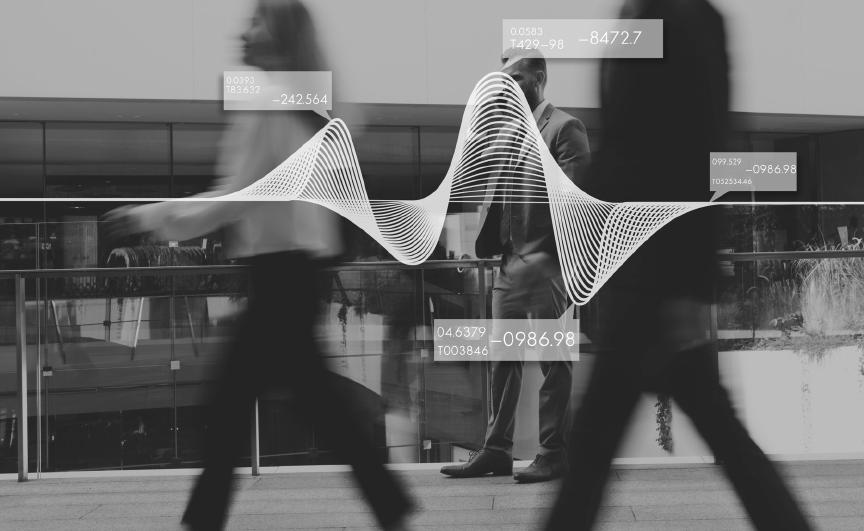
Social media is a window to the rest of the world. Every day, billions of people log in to Instagram, Facebook, TikTok, and other social media platforms to consume news, be entertained, and connect with their friends, colleagues, and relatives. Their experiences on these platforms are governed by highly sophisticated algorithms that push content and suggest possible connections with other users.
Each social media platform has its own proprietary algorithm that mines the constantly updating feed for important data. While these companies keep the inner workings of their algorithms closely guarded, it’s easy to deduce that they rely on some type of recommendation system. This is how you can easily discover fresh videos that cover your interests on YouTube or find and connect with long-lost friends on Instagram.
In this article, we will cover how text-based recommendations in social media work and how to use them to your brand’s advantage.
The need for contextualized recommendations in social media
People born before 2000 likely remember when " The Algorithm " didn’t dictate our digital lives.” By 2008, when Facebook and Twitter were the most exciting websites on the internet, social media recommendation engines were still in their infancy. If suggested content was even a feature, the execution was relatively bare-bones.
At that time, rather than being served content based on the calculations of a predictive algorithm, users curated their own feeds by following, liking, and subscribing to the creators whose output they enjoyed.
This subscription-based model is falling out of fashion and being replaced by algorithms. Thanks to advances in artificial intelligence (AI), machine learning (ML), and natural language processing (NLP), recommendation engines are now able to predict a user’s reaction to content even before they see it.
User activity, past behavior, demographic details, buying habits, and engagement patterns are all factors that influence the algorithm’s content delivery pattern.
Importance of personalized content recommendations
When social media platforms used the subscription model, users saw very little irrelevant content in their news feeds. However, they couldn’t see any content they may have been interested in but haven’t subscribed to.
Implementing an effective social media recommendation engine solves this problem. It pairs users with content that matches their interests, giving them a more personalized user experience (UX). It also helps the discovery process, where platform users are served a steady supply of fresh content from outside the pages and channels they already follow.
Text-based recommendations in social media are central to prolonging screen time and encouraging engagement. Even video and image-based platforms like YouTube and Instagram can mine the unstructured meta-data of every post and user profile, which is then analyzed and used to make predictions about how people would potentially react to a particular piece of content.
How recommendation systems personalize content for social media users
Every user’s profile is unique. For example, two individuals with similar demographics like age, gender, and location might have very different-looking Instagram feeds. This is because every user’s profile contains signals and metadata that help recommendation engines find content just for them.
Here are a few examples:
-
Engagement rates, such as likes, comments, and shares.
-
Relevance to user interests based on keywords and hashtags.
-
Timing of the post and post frequency rate of the uploader.
-
Recency that prioritizes newer posts over older ones.
-
User interactions, such as click-throughs and accounts followed.
-
Profile statistics, like the number of followers and reach.
-
Proximity based on location data.
-
Virality, or how many other people have already seen and interacted with the content.
These are only some of the most prominent metrics considered by social media recommendation engines. Their complete scope is far more complex and continuously evolving with each software patch and upgrade.
How user activity shapes social media recommendations
When a recommendation system makes predictions about what type of content a social media user might enjoy, it doesn’t make them in a vacuum. It relies on preexisting data about the user, converting it into text analytics and extrapolating from there.
This can be explicit data (provided by the user) or implicit data (automatically generated through user activity). Let's look at the impact of user activity on social media recommendation engines.
Interest and connections
User interests and social connections shape context. This is a form of implicit data gathered by tracking the type of content users engage and interact with. Their social connections also influence the recommendation engine.
For example, if a person has five friends in their network interested in pottery, the recommendation engine could suggest pottery-related content to that person even if their activity hasn’t demonstrated a direct interest in the subject.
Real-time adaptation
Recommendation engines recalibrate and refine results by performing real-time analysis of user activities and trending topics.
For example, posts about football will be on most people’s feeds during Super Bowl Sunday because it is the biggest trending topic of the day. The recommendation system suggests more related content if a user consistently interacts with posts under a specific topic.
Techniques used for social media recommendations
Let’s take a peek behind the curtain and see what’s really going on when a recommendation engine suggests content on social media.
Collaborative filtering
Collaborative filtering relies on other people’s data to create recommendations for the active user.
Here’s a simple example. A fashion-conscious shopper follows the brand pages for Rolex and Versace, while another user in the same demographic follows Rolex, Versace, and Gucci. The recommendation engine will detect this similarity in previously followed pages and recommend Gucci to the first user.
Collaborative filtering is best for large groups of users and items because it uses a bigger dataset to make inferences and predictions.
Topic modeling
From post captions to comments, nearly every piece of content on social media is accompanied by some form of text. Topic modeling is an NLP technique that identifies recurring themes, concepts, and topics that occur within a single document or a collection.
Using topic modeling, the new generation of AI- and NLP-powered recommendation engines can reliably classify content into subjects that can then be matched to a subset of users.
How recommendation systems keep social media feeds fresh
Our entire digital lives are largely powered by recommendation engines. The sheer amount of content on social media platforms is overwhelming to navigate without some sort of compass, which these text-based recommendation systems can provide.
Let’s examine the two most important ways social media recommendation engines improve a platform’s UX.
Provide tailored news feeds and advertisements
Discovering relevant content no longer requires active effort from users. By analyzing a combination of implicit and explicit data, recommendation engines can deliver a steady stream of content to any feed, even if the user’s activity is minimal.
Relevant content constantly streams in, causing users to spend more time on the platform, and providing the recommendation system with even more data to refine its results in a continuous improvement cycle.
Balance personalization with diversity
One of the most common criticisms of social media platforms is that their algorithms promote the creation of echo chambers. But this is not entirely true.
Recommendation engines are instrumental in helping users discover new content, occasionally providing suggestions outside the user’s regular list of content creators and news sources.
Dynamic content filtering and ranking strategies
For many businesses, social media has become their first point of contact with their target audience. If you want to increase brand awareness and attract new customers through these platforms, your brand’s posts need to break through the clutter. To do that, you need to know how to play by the rules of the platform’s recommendation engine.
Though each platform’s algorithm functions slightly differently from the others, there are a few universal best practices to keep in mind regardless of whether you’re posting on Instagram, TikTok, LinkedIn, or X:
-
Recency: Newer posts receive more attention.
-
Popularity: Responding to popular posts helps connect your brand profile to a topic.
-
Engagement: Design posts to invite engagement since that increases the reach.
Every interaction or post on social media provides the recommendation engine with another data point to better match your brand to its target audience.
Limitations of social media recommendation engines
There have been some justified concerns about how social media algorithms affect our online experience. Thankfully, every new generation of recommendation engines is accompanied by corresponding increases in technological safeguards.
Here are the most common drawbacks of social media recommendation engines and what can be done to reduce their impact.
-
Privacy
Users often upload personal and private information to their social media profiles and are understandably protective. Allowing users to choose what data they wish to share with the platform lets them feel comfortable with having their activity tracked.
-
Scalability
As the number of users on a social network grows, it becomes harder for a simple content-based or collaborative filter system to keep up. Newer recommendation systems use a hybrid filtering method, taking the best aspects of content-based and collaborative filtering to develop a scalable and efficient solution.
-
Bias
While recommender systems are remarkable in operating with almost complete independence, a certain level of human intervention is still needed. AI software is only as good as the data it was trained on, which means preexisting biases in the dataset will be amplified. A level of oversight by human operators can ensure that the recommendation engine is only training on verified, statistically sound data.
What success looks like for text-based recommendations in social media
Today, most millennials and Gen Z adults spend more time scrolling social media than watching television. A report published in Forbes showed that the average internet user spends 30% more time on social media than watching TV.
This gap is a testament to the effectiveness of social media recommendation engines in delivering content that users want to consume.
Let’s look at how one of the biggest social media platforms pulled this off.
YouTube
YouTube’s algorithm has evolved significantly over the years. It was one of the earliest platforms to incorporate classifiers, which flagged inappropriate content and filtered it out.
Today, YouTube uses many different metrics, including watch time, customer feedback forms, a robust classification system, and ML techniques to deliver must-watch recommendations for all users.
Future directions and trends for social media recommendation systems
The rise of AI- and ML-powered recommendation engines with NLP capabilities is exciting and full of potential.
As recommendation engines become even more refined and powerful, we could start seeing cross-platform integration and decentralized recommender systems that mine data from all over the internet, not just social media.
Get a glimpse into the future of business communication with digital natives.
Get the FREE report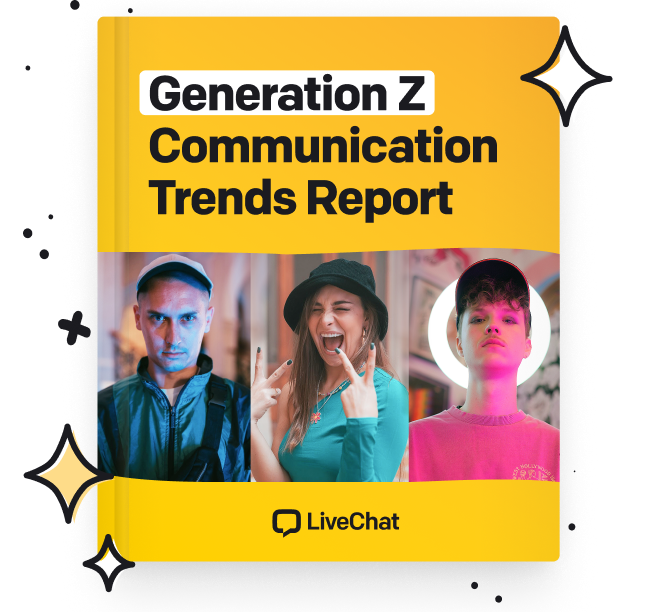