Text Clustering in Recommendation Systems: Personalizing Recommendations with Similarity Analysis
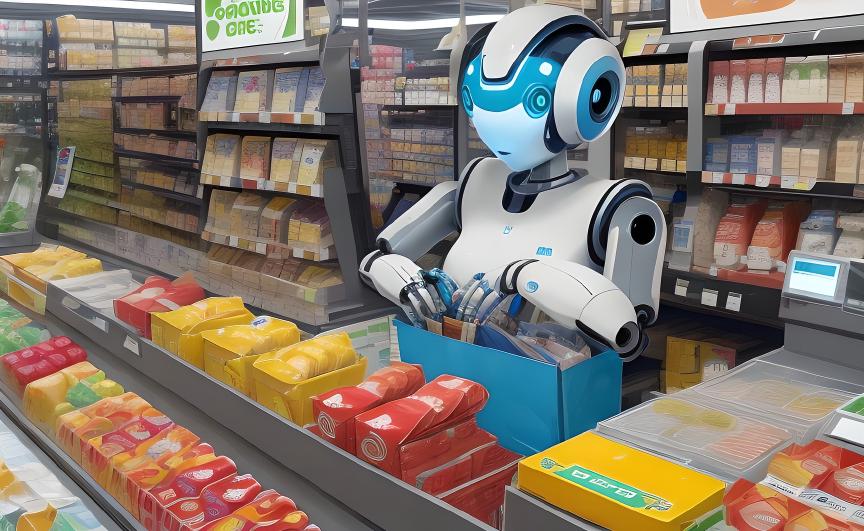
Ever felt like your favorite online platform just 'gets' you?
That uncanny knack Spotify has for introducing you to your next favourite song, or how Netflix seems to know the exact series you'd binge-watch next?
It's not serendipity; it's sophisticated technology in action.
At the heart of these tailored experiences lie recommendation systems powered by text clustering and similarity analysis.
These systems sift through vast amounts of data, grouping similar content and analysing patterns to curate suggestions that resonate with your unique preferences.
It's why every time you log in, it feels like the platform was designed just for you.
This blog delves into the intricate dance of text clustering in recommendation systems, shedding light on how digital products seamlessly align with our interests.
Discover the science behind the digital platforms that seem to understand you better than you understand yourself.
The Evolution of Recommendation Systems
Back in the day, recommendations were simple. Recall those early 2000s e-commerce sites? Products were showcased based on what everyone else was buying.
The mantra was straightforward: if it's popular, it must be good. But as the digital landscape matured, so did the expectations of users. They no longer wanted what everyone else had; they craved a unique digital experience tailored to their preferences.
Enter the era of personalization. Digital platforms recognized the immense value in curating content that resonated with individual users.
By doing so, they enhanced user engagement and significantly boosted their sales and retention rates. Consider an e-commerce giant like Amazon. Instead of merely showcasing bestsellers, it began to analyze user behavior, past purchases, and browsing patterns.
The result?
A homepage uniquely crafted for every user, displaying products they're more likely to purchase.
This shift from generic to personalized wasn't just a trend but a necessity. In an age of fleeting attention, offering customized content ensures users remain engaged and feel valued.
But how do platforms decide what's relevant to each user? The answer lies in the intricate world of text clustering, a technique revolutionizing recommendation systems.
The Basics of Text Clustering
Imagine you've got a jumbled box of puzzle pieces. Text clustering is akin to sorting these pieces by colour or pattern, making it easier to see the bigger picture.
But in the realm of digital data, this process is far more intricate than it appears. Text clustering is a method used to group similar pieces of textual information based on their content. This is achieved through algorithms that measure the similarity between different texts.
Often, text clustering isn't just a standalone software but is embedded as an integral part of larger systems.
Many digital platforms, especially those dealing with vast data, incorporate text clustering algorithms to organise and categorise information. The technology behind it is fascinating.
Given the complexities of the English language, where a single word can have multiple meanings based on context, the task is challenging. For instance, the word "bank" can refer to a financial institution or the side of a river.
Algorithms use context, co-occurrence, and other linguistic cues to discern the correct meaning.
Consider a news aggregation platform. With thousands of articles published daily, how does it categorise them into coherent sections?
Text clustering analyses the content, identifies patterns, and groups similar articles together, ensuring users easily find relevant stories.
How do recommendation systems personalize suggestions?
By analyzing user behavior and preferences, recommendation systems craft a digital experience tailored for each user. Text clustering and similarity analysis drive a significant part of this personalization.
When a user interacts with a platform, they leave behind digital footprints. These interactions, whether clicking on a product, reading an article, or even pausing to view content, are invaluable data points.
Recommendation systems harness this data, using text clustering to identify patterns.
For example, the system recognizes this preference if you've been browsing eco-friendly products on an e-commerce site.
The next time you visit, it might recommend sustainable brands or highlight green initiatives, ensuring the content aligns with your interests.
But it's not just about past behavior. Similarity analysis dives deeper, comparing the content of items to discern how alike they are.
If you've ever wondered why after reading an article on ancient Greek history, you're recommended a piece on Roman architecture and it's similarity analysis at work. Both articles might share keywords, themes, or concepts, making them contextually similar.
In essence, recommendation systems are like digital butlers, always a step ahead, anticipating your needs and curating content that resonates with your preferences.
The magic behind this impeccable service? A blend of text clustering and similarity analysis.
Why It Matters in Today's Digital Age
In today's digital age, the sheer volume of information available at our fingertips can be overwhelming.
Amidst this deluge, similarity analysis stands as a beacon, ensuring the content we encounter is relevant and resonant. It's not just about sifting through data; it's about refining our online experiences, making them more fulfilling and satisfying.
When we search for a topic, read an article, or explore a new interest, similarity analysis ensures that the content presented aligns with our intent and preferences. It recognises patterns, understands context, and draws connections between seemingly disparate pieces of information.
The result?
A tailored digital journey, where every piece of content feels like the next logical step, enhancing our understanding and satiating our curiosity. In essence, similarity analysis transforms the vast digital landscape into a personalised map, where every path leads to discovery and enlightenment.
While this tailored experience enriches our personal online journeys, one can't help but ponder the potential advantages for the other side of the screen. How might businesses harness the power of similarity analysis to their benefit?
Similarity Analysis for Business
For small business owners navigating the vast digital landscape, harnessing the power of similarity analysis can be a game-changer.
At its core, similarity analysis and text clustering offer a way to make sense of vast data, drawing connections and identifying patterns that might otherwise go unnoticed.
One of the most tangible benefits for businesses is the ability to offer personalized recommendations. Imagine a customer visiting your online store.
They browse a few products and add one to their cart but leave without purchasing. With similarity analysis, they could be presented with products that align closely with their previous browsing behavior the next time they visit.
It's not just about showing them what they looked at before, but introducing them to new products they're likely interested in. This level of personalization can significantly enhance the user experience, making customers feel understood and valued.
Beyond just product recommendations, text clustering can help businesses organize their content more effectively.
Whether it's blog posts, product descriptions, or customer reviews, clustering similar content together can make it easier for customers to find what they're looking for, enhancing their overall experience with your brand.
Moreover, recommendation engines, powered by similarity analysis, can be integrated into various aspects of a business's digital presence.
From email marketing campaigns to social media adverts, businesses can ensure that the content they're putting out is tailored to the individual preferences of their audience.
The Benefits for Tech Companies
The rise of similarity analysis and text clustering isn't just a boon for small businesses; it's also opening up opportunities for tech companies.
As the demand for personalized experiences grows, tech enterprises have the chance to delve deeper into the intricacies of similarity structures and pattern analysis.
By doing so, they can develop computational models that are more refined, accurate, and adaptable to the ever-evolving digital landscape.
Tech companies can harness these advanced models to create tools and platforms explicitly tailored for small business owners.
These tools could offer more nuanced insights into customer behavior, enabling businesses to anticipate needs and preferences with even greater precision. Furthermore, as these computational models become more sophisticated, they can identify subtler patterns and structures in data that were previously overlooked.
For tech enterprises, this means the potential to develop a new generation of products that are more powerful and intuitive.
By integrating advanced similarity structures and leveraging pattern analysis, they can offer solutions that make the complex world of digital data more accessible and actionable for small business owners.
As technology advances, the bridge between sophisticated data analysis and everyday business operations becomes more robust, paving the way for a future where data-driven insights are within reach for businesses of all sizes.
Content-Based vs. Collaborative Filtering
Diving into the realm of recommendation systems, two dominant approaches emerge content-based and collaborative filtering.
Content-based systems primarily analyze the attributes of items. For instance, a music streaming service might consider genres, artist types, or beats per minute.
The system recognizes this preference and recommends other jazz tracks if you frequently listen to jazz. The strength here lies in its specificity; it's directly aligned with user preferences based on their interactions with content.
On the flip side, collaborative filtering zeroes in on user behavior. It operates on the premise that if two users agree on one issue, they will likely agree on others.
So, if you and another user enjoyed the same ten books, and they read an eleventh book and loved it, the system would recommend it to you. Its power is in the collective intelligence of users.
Platforms like Amazon often employ this, suggesting items with "Customers who bought this also bought..."
Technically, content-based systems rely on item attributes and use them to build user profiles, while collaborative filtering uses user-item interaction matrices, often leveraging techniques like matrix factorization.
When applied aptly, both models enhance similarity analysis, ensuring recommendation systems are precise, relevant, and dynamic. Choosing between them hinges on the nature of the application and the available data.
In the vast digital expanse, where data is generated at an unprecedented rate, businesses face a daunting challenge: making sense of this colossal data to offer meaningful experiences to users.
Enter text clustering, a technique that's proving invaluable in this endeavor.
The Role of Text Clustering in Personalization
The challenge for businesses is to harness this data effectively, ensuring it translates into meaningful user interactions. Text clustering stands at the forefront of this endeavor, offering a method to streamline and personalize content.
By analyzing and organizing data, this technique refines the user experience, making it more tailored and relevant.
Making Sense of User Data
The sheer volume of data available can be both a boon and a bane. While it offers a treasure trove of insights, the challenge lies in sifting through it to extract what's relevant.
Without the right tools, this data can be like a needle in a haystack, making it harder for businesses to find what they truly want. Text clustering provides a solution by organising this data, and grouping similar pieces of information together.
For platforms with millions of users, the stakes are high.
Each user expects a tailored experience, a digital journey that feels crafted just for them. How can platforms meet these expectations?
By employing text clustering to analyze user data, platforms can discern patterns and preferences that are unique to individual users.
This ensures that recommendations are personal and relevant, striking a chord with the user's interests and needs.
Enhancing User Experience
But personalization isn't merely about catering to likes; it's about anticipating love. It's about understanding a user so well that the platform can introduce them to content they didn't even know they'd adore.
This level of refinement in recommendations, powered by text clustering, ensures users find what they're looking for faster, leading to a more enjoyable and satisfying experience.
The benefits of such personalization are manifold. For starters, it builds a rapport with customers. In an age where users are inundated with choices, personalization stands out, making users feel valued and understood. This fosters loyalty, a trait that's becoming increasingly crucial.
Today's users are loyal to quality brands, not just their products. They seek consistency in experience, and brands that deliver this win their trust.
This loyalty translates to tangible business benefits.
A loyal and returning customer is a goldmine for businesses. They bring in consistent revenue and act as brand ambassadors, spreading the word and bringing in new users. In contrast, acquiring new users is essential but comes with rising costs.
The investment required to bring in a new user often outweighs the immediate returns. Hence, in the long run, it's more feasible for businesses to delve into their existing data, identify patterns, and enhance the experience for their current user base.
Real-World Impact: Stories of Success
Text clustering, a subset of machine learning, has become an indispensable tool for platforms aiming to refine user experiences.
By grouping similar pieces of textual data, it aids in discerning patterns and preferences, which in turn, informs recommendation engines.
Meta (formerly Facebook) utilizes text clustering to categorize vast amounts of content shared daily. It can identify trending topics and user interests by analyzing posts, comments, and shared links.
This data informs the content that appears on individual news feeds, ensuring users see posts most relevant to their preferences.
Netflix employs a combination of content-based filtering and collaborative filtering.
While content-based filtering uses text clustering to categorise movies and series by themes, actors, and directors, collaborative filtering recommends content based on what similar users have watched. This dual approach ensures users receive tailored content suggestions, enhancing binge-watching sessions.
Spotify uses text clustering to categorize songs by lyrics, genre, and artist type.
Their 'Discover Weekly' playlist is a testament to the efficacy of their algorithms, offering users new songs that align closely with their listening history.
Shopify leverages text clustering to help merchants organize their product listings.
Analyzing product descriptions, reviews, and metadata can suggest product groupings or themes, aiding in more intuitive site navigation for shoppers.
HubSpot, a CRM platform, employs text clustering to analyse customer feedback, emails, and chat logs.
This helps businesses identify common customer pain points or frequently asked questions, enabling them to refine their customer service approach.
A notable implementation is Spotify's 'Wrapped' feature. Using text clustering and other recommendation engine techniques, Spotify provides users with a year-end summary of their listening habits, top genres, and artists.
This enhances user engagement and promotes the platform's prowess in data analytics and personalization.
These platforms harness text clustering and recommendation engines to create a more personalized, intuitive user experience. This focus on individual user preferences enhances user satisfaction and drives platform growth and loyalty.
The Road Ahead: Challenges and Opportunities
The increasing reliance on personalized recommendations, underpinned by text clustering and similarity analysis, presents challenges and opportunities for businesses in the digital age.
As platforms like Meta, Netflix, and Spotify harness these techniques to curate tailored user experiences, concerns arise about the potential pitfalls of over-personalization.
Firstly, there's the risk of creating echo chambers.
By consistently serving users content that aligns with their existing preferences, platforms may inadvertently limit exposure to diverse perspectives and new experiences.
This can lead to a narrowed worldview, stifling creativity and innovation.
Secondly, the accuracy of data-driven personalization is contingent on the quality and breadth of the data collected. Incomplete or biased datasets can lead to misinformed recommendations, potentially alienating users. Moreover, the ethical considerations of data collection and usage come to the fore.
Users may become wary of platforms that appear to know too much, leading to privacy and data security concerns.
Lastly, businesses face the challenge of balancing automation and human touch. While algorithms can process vast amounts of data efficiently, they lack the nuanced understanding and empathy inherent to human judgment.
Over-reliance on automated recommendations might result in missed opportunities to connect with users on a deeper, emotional level genuinely.
Wrapping Up
The rise of personalized recommendations, driven by text clustering and similarity analysis, has transformed user experiences on digital platforms. While platforms like Spotify and Netflix have harnessed this technology to curate content that resonates with individual preferences, challenges persist.
Over-personalization risks creating echo chambers, limiting users' exposure to diverse content. The efficacy of these recommendations hinges on the quality of data collected, with biased datasets potentially leading to misinformed suggestions.
Furthermore, the balance between automation and human touch remains crucial.
As technology evolves, businesses must navigate these complexities, offering enriched, relevant experiences without compromising user trust or data integrity.
Get a glimpse into the future of business communication with digital natives.
Get the FREE report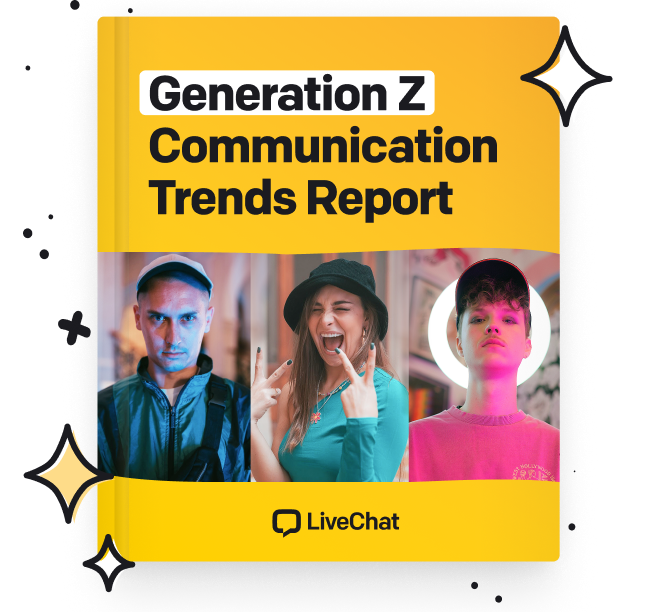