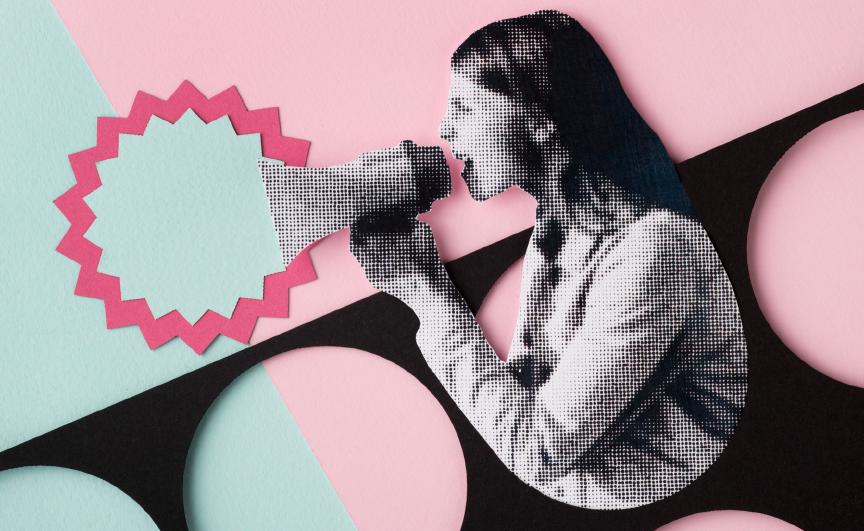
Do you know how your customers feel about your service/product? If not, you won’t meet their expectations or retain them for the long term.
Luckily for you, customer sentiment analysis can help you track customer sentiment about, well, customer feedback. You can make informed decisions and improve customer experience, gaining a competitive advantage over your peers in the industry. Investing in customer sentiment analysis can double your revenue in 36 months.
Text mining is a sentiment analysis tool that uses machine learning (ML) and natural language processing (NLP) to measure customer sentiment by extracting useful information from unstructured data like customer communications to facilitate customer sentiment analysis.
It is an underutilized but powerful approach to feedback analysis that can become a key differentiator for your business and contribute to its success.
The power of text mining
Data is the most valuable and powerful currency that you have at your disposal. But in a world where data is produced and consumed every waking hour of every day, most of it is unstructured and, therefore, unusable.
You might be surprised to know that 80% of data generated globally is unstructured, even though it can potentially become the transformative force your business needs to make headway in an increasingly competitive landscape.
Text mining can be the technological juggernaut that unlocks and harvests the power of otherwise unusable text data for your business. Its superpower lies in its quantitative ability to process vast volumes of unstructured text data in your customers’ digital footprint.
An autonomous process, text mining analysis is a data mining technique that solely analyzes text-based data. This includes social media posts, customer interactions with chatbots/customer care personnel, discussions in online forums, email tickets, feedback forms, and any data stored in written format.
Because text-based data is highly subjective, it’s a surefire indicator of your customers’ sentiments regarding your product/service, overall brand perception, and expectations. That’s why text mining to extract insights from textual customer data is essential for your business.
Analyzing text data can also help you streamline your targeted marketing practices, conduct in-depth market research, understand negative sentiment, build better customer redressal systems, and improve customer satisfaction scores.
You can do all this with ease because text data:
-
Is plentiful.
-
Is easily accessible.
-
Is simple and inexpensive to store.
-
Contains trends/patterns that may not be easily identifiable in other data sources.
So, you must leverage text mining for customer sentiment analysis — and for this, you need to learn how to make it a part of your opinion analysis process.
Leveraging text mining in sentiment analysis
Structured data is a prerequisite for opinion analysis. That’s why text mining is the first and the most necessary step to flag off customer sentiment analysis.
Text data mining gathers data, pre-processes it, and performs content analysis to structure it. It’s a complex data mining technique heavily dependent on AI-ML and NLP for execution.
The process can facilitate a rule-based sentiment analysis approach that utilizes classifiers determined by your marketers to categorize customer sentiments. It could also be used with ML algorithms that can empower computational models to discern what your customers think of your brand/offering.
Both models have pros and cons. For foolproof text mining, use a hybrid approach combining rule-based and ML-based customer sentiment analysis.
You can then choose to perform sentiment analysis in the following ways:
-
Aspect-based to determine which aspects of your offering the customer likes and which need improvement.
-
Intent-based to understand how interested or disinterested your customers are in new products, discounts, value-added services, etc.
-
Emotion-detection to discover how your customers felt while writing the review/talking to a chatbot, etc.
-
Fine-grained Scoring to mark your customers’ behavior on a scale of positive to negative.
As you move from fine-grained scoring to emotion detection in sentiment analysis, insights' accuracy increases with the analysis's granularity. That’s why text mining makes sentiment analysis highly precise and consistent.
Just remember that both processes must work in tandem to become useful to you.
This means you must integrate text data mining techniques with sentiment analysis algorithms. You can also consider deploying an end-to-end software-as-a-service (SaaS) solution that utilizes text mining for customer sentiment analysis.
Unraveling customer insights
Both rule-based and ML-driven text data mining models can be trained to analyze customer sentiments as positive, negative, or neutral. A combination of predictive technology, linguistic analysis, and advanced NLP can train computational systems to identify the intent of words used by customers when talking about the brand/product.
So, the application of text mining in sentiment analysis goes beyond structuring data. It can make a preliminary reveal of the underlying sentiment of text-based data that customer sentiment analysis can build upon.
Sentiment analysis algorithms can study the data from text mining and provide deeper insights that can guide your business strategy.
For example, if your sentiment analysis findings reveal positive sentiments about a newly launched product, you can consider adding similar products to your repertoire. Negative responses should indicate the need to improve to reduce customer churn or discontinue the product.
Uncovering NLP techniques used for deeper understanding of text data
At this point, you must be wondering how text mining for sentiment analysis gets so much done. The answer lies in NLP.
In the preprocessing stage, text data mining relies on the following NLP methodologies to prepare customer data for content analysis.
Tokenization
This is the first step in NLP that breaks up sentences into smaller units or tokens. Tokens can be words, subwords, or characters based on the input prompt. Tokenization makes written words legible to computational models.
Parsing
Parsing is an NLP methodology that checks for grammatical syntax in tokenized texts. It can be used for information retrieval, text classification, and sentiment analysis.
Lemmatization and stemming
Lemmatization converts words into their respective roots and groups similar words together. The process is contextual and enables ML algorithms or rule-based systems to understand words so that these can be processed later for sentiment analysis.
In contrast, stemming works by removing the characters from the end of words to convert them to root words.
Different text mining techniques can benefit from either of the two processes.
Stop removal
This is the process that removes unnecessary/unimportant words from a dataset. Since it reduces data size, it helps text mining algorithms do their work more efficiently and quickly.
All these NLP methodologies contribute to text data mining. Trained using big data sets, neural networks, and deep learning techniques, these NLP techniques can be taught to identify polarities and cultural and historical nuances of words.
Although it still has a long way to go before it can shed its Sheldon Cooper-esque perception of sarcasm, advanced text mining can eliminate double negatives or interpret the contextual meaning of words.
So, NLP methodologies, supplemented by AI, ML, and other innovations, make text mining what it is.
Real-world applications of text mining in customer sentiment analysis
Unlike traditional customer surveys, text analytics tools such as this provide an intimate glimpse into customer experience. Because it considers all text-based customer-generated data, it works with a large data set and can provide a holistic and precise understanding of customer sentiments.
It’s like looking into a mirror and checking the truth for yourself.
A team of researchers from Oklahoma State University utilized text mining to perform customer sentiment analysis of top-selling speakers on Amazon. Product reviews were used as the primary source of text-based customer data. These reviews were then subjected to customer sentiment analysis.
The experiment's results allowed researchers to identify features that customers loved and hated in five speaker models of different brands.
You could also perform a similar market research experiment before launching a new product. By leveraging text mining for sentiment analysis, you can discover product features favored by your target audience and use them in your product development.
However, this is only one of the many possibilities of text mining in customer sentiment analysis. It has endless uses, as discussed above. You just have to trust the process and use it to your advantage.
The need for a seamless integration of text mining
Developing sophisticated AI-driven text mining tools for customer sentiment analysis may not be your first choice because executing it might be impractical. That’s why you must consider integrating third-party text mining solutions into your existing sentiment analysis architecture.
Dedicated SaaS solutions for customer expectation management offer sentiment analysis as a core feature. You can also deploy NLP APIs built for text analysis.
Another option you can opt for is creating AI-driven automated workflows. You will need a sound knowledge of AI to make text mining workflows.
Step up your customer sentiment analysis game with text mining
By now, you must have realized that text mining shares a deeply symbiotic relationship with sentiment analysis. You can unlock the hidden potential of customer sentiment analysis when you juxtapose it with text data analysis for all the reasons discussed in this article.
Bonus: It’s affordable and yields an impressive ROI.
Now’s the time to integrate text mining into your customer sentiment analysis process to boost your customer service through actionable l insights. Customer reviews can also influence prospective buyers and their decisions to associate with your brand. To gain customer loyalty, you need to improve customer experience with the help of sentiment analysis.
Get started today.
Get a glimpse into the future of business communication with digital natives.
Get the FREE report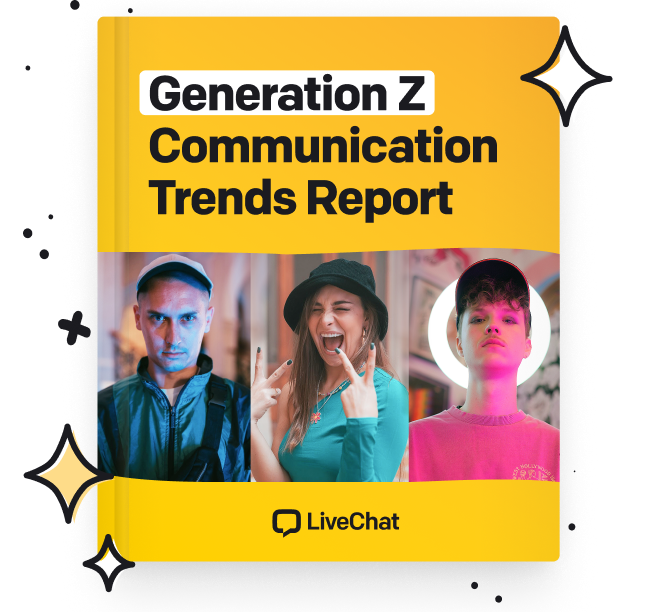