Linguistic Identity Detection Techniques: From Statistical Models to Machine Learning
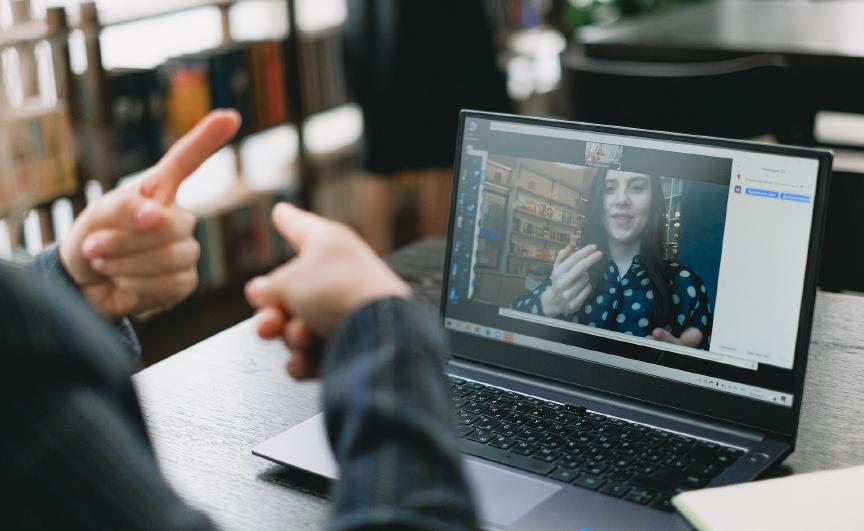
Ever wondered how platforms discern between British English and American English?
Or how can they tell if a text was written by a native speaker or not?
That's the magic of linguistic identity detection. This intricate technology is more than just a behind-the-scenes player; it's a game-changer in today's digital age.
Consider the world of online customer support. When you type a query into a chatbot, it often tailors its response based on the linguistic nuances of your message. Whether you're using colloquialisms from the streets of London or the slang of New York, the system recognizes and adapts.
In this article, we dive deep into the mechanics of this fascinating field. From the foundational statistical models that paved the way to the cutting-edge machine learning algorithms that are setting new benchmarks, we'll explore the journey of how technology is continually refining its understanding of our diverse linguistic identities.
The Importance of Linguistic Identity
For business:
Linguistic identity holds paramount significance in business, security, and technology.
For businesses, understanding linguistic nuances can be the difference between resonating with a target audience and missing the mark entirely. Tailored marketing campaigns, customer support, and product localization all hinge on a deep understanding of linguistic identity.
From a security perspective, linguistic identity detection acts as a robust layer, identifying potential threats or fraudulent activities based on linguistic patterns. It's not just about recognizing a language but discerning subtle differences that might indicate deceit or impersonation. Technological advancement, too, is deeply intertwined with linguistic identity.
As artificial intelligence and machine learning evolve, their ability to accurately detect and adapt to linguistic nuances becomes a benchmark for sophistication.
In essence, linguistic identity is not just about language; it's a cornerstone for effective business strategies, robust security measures, and the next frontier in technological innovation.
For culture:
Linguistic identity detection is not merely a marvel of modern technology; it's a profound instrument that deeply connects with the essence of our multifaceted cultures. It acts as a bridge, enhancing communication clarity, and stands as a vigilant protector against deceit and misrepresentation.
Delving into the sophisticated domain of language technology, linguistic identity detection emerges as a beacon of hope. It transcends the simple identification of words. It's a passionate journey to capture the essence, trace the origins, and applaud the unique attributes of a text. Every word, phrase and intricate nuance is meticulously dissected, comprehensively depicting the writer's linguistic heritage and distinct style.
But why is this so pivotal?
Reflect on the tragic events of the Holocaust, where countless lives were lost, and several Hebrew dialects vanished with them. These dialects were more than just modes of communication; they were the carriers of stories, traditions, and a rich cultural history. Now, imagine the power of linguistic identity detection in such a context. It could potentially recognize and, in some ways, resurrect elements of these lost dialects, preserving cultural fragments that were almost obliterated. By digitally archiving remnants of these dialects, we safeguard them for posterity and gain insights into their unique linguistic intricacies.
In our interconnected world, where cultures continuously merge and communication is of utmost importance, precisely identifying and valuing linguistic identities is indispensable. It's not just about technological prowess; it's a heartfelt commitment to cherish, safeguard, and elevate the diverse tapestry of our global cultures.
Rights and Permissions in Linguistic Identity Detection
In the realm of linguistic identity detection, the issue of rights and permissions emerges as a significant concern. The technology's ability to discern linguistic nuances is profound. However, with this capability comes the challenge of ensuring that data is accessed and processed ethically and legally.
Companies must obtain explicit permission to analyze and use linguistic data, especially concerning personal or sensitive information. The challenge intensifies when considering diverse global data privacy regulations, such as Europe's GDPR. Furthermore, there's the ethical dimension: even with permissions in place, the potential misuse of linguistic data to stereotype or discriminate against certain language groups poses a real threat.
While linguistic identity detection offers immense benefits, entities must navigate the intricate maze of rights and permissions. Ensuring compliance and ethical application is not just a legal mandate but a responsibility to the diverse linguistic communities they serve.
Evolution of Detection Techniques
From simple rule-based systems to advanced algorithms, the journey of linguistic identity detection has been revolutionary.
Statistical Models in Linguistic Identity Detection
Language detection techniques have seen a marked progression. Beginning with basic rule-based systems, the field has advanced to employ intricate algorithms. Early methods were anchored in static rules and dictionaries, which, while functional, had their limitations in adaptability. The advent of statistical models ushered in a new phase. These models, leveraging word frequencies and patterns, enhanced detection accuracy. The true paradigm shift, however, was heralded by machine learning. Trained on extensive datasets, these algorithms pinpoint nuanced linguistic elements with unmatched precision. Neural networks, an advanced facet of machine learning, have further honed this capability, detecting complex language structures.
As artificial intelligence integrates into the field, language detection is poised for further advancements, aiming for unparalleled accuracy and flexibility. The continuous advancements in this sector highlight its critical role in today's globally connected environment.
Basics of Statistical Models
Statistical models, often regarded as the foundational pillars in linguistic detection, operate on the principles of probability and discernible patterns. By meticulously examining the frequencies of words, the sequences in which they appear, and recurring patterns, these models deduce the linguistic identity of a text. Their methodology is grounded in data-driven analysis, where vast amounts of textual information are processed to derive meaningful insights about language structures and tendencies.
Pros and Cons
Statistical models, owing to their data-centric approach, are inherently robust. Their strength lies in their ability to provide consistent results based on established patterns. However, this strength can also be a limitation. Languages are dynamic, constantly evolving and adapting. Being anchored in historical data, statistical models might struggle to keep pace with rapid linguistic changes, making them less flexible in specific scenarios.
Real-world Applications
The influence of statistical models is pervasive, often in areas we might overlook. Consider the predictive text feature on smartphones. When typing a message, the suggested words are often the result of statistical models analyzing common word sequences. Another prime example is email platforms. The way they filter out unsolicited emails, or spam is primarily based on statistical models identifying patterns typical of unwanted messages. Silently operating in the background, these models enhance user experience and streamline digital communication.
Machine Learning, Language processing, and Linguistic Identity Detection
What is Machine Learning?
Machine learning is a paradigm where machines are trained to extract knowledge from data, mirroring how humans assimilate lessons from experiences. It's a cornerstone in computational linguistics, enabling systems to understand and process human language in previously unimagined ways.
Machine Learning in Linguistics
Machine learning algorithms dive deep into extensive textual data within the vast expanse of linguistic identity. They analyze and grasp the subtle nuances of language groups, distinguishing between similar languages and even dialects. By doing so, they can accurately identify the language of new, previously unanalysed data, making them invaluable in tasks like language identification and natural language processing.
Comparing Traditional and Modern Approaches
The discourse often centers on the effectiveness of age-old models compared to modern machine-learning techniques. Machine learning, an integral part of artificial intelligence, is heralded for its unmatched capabilities in the realm of linguistic identity detection. Its edge? The flexibility to adapt, the ability to scale, and the prowess to handle enormous datasets. Such traits empower these algorithms to pinpoint linguistic identities, even differentiating native language nuances from non-native ones, with remarkable accuracy.
Advantages of Machine Learning Techniques
Machine learning techniques are tailored for the digital era. Their adaptability, scalability, and data-handling capabilities make them indispensable, especially when dealing with diverse social identities and the complexities of human language.
Challenges and Limitations
Yet, perfection remains elusive. These algorithms demand vast datasets for training. Their operations, often likened to a 'black box', can be opaque, leading to trust issues. Furthermore, if not calibrated meticulously, they risk overfitting, where they become too attuned to the training data, compromising their generalization capabilities. Notably, neural network-based models, while powerful, can be particularly susceptible to such pitfalls.
Comparing Statistical Models and Machine Learning
Efficiency and Accuracy
Historically, statistical models, anchored in probability and discernible patterns, have been the cornerstone of linguistic identity detection. Their steadfast reliability, refined over decades, has made them indispensable in early computational linguistics. Yet, as we transition into the age of data-driven decisions, machine learning is emerging as a formidable contender. Companies like Google and IBM have leveraged machine learning to enhance language identification in their products. The edge machine learning possesses lies in its precision, especially when nurtured with vast, diverse datasets. For instance, once primarily based on statistical methods, Google Translate thrives on neural machine translation, showcasing superior accuracy in real-time translations. This shift to machine learning is not just a technological transition but a testament to its superior efficacy in contemporary applications.
Scalability and Adaptability
Machine learning's prowess is most evident in its scalability. In today's data-abundant world, companies like OpenAI and DeepMind employ machine learning to sift through and learn from petabytes of data. This scalability is coupled with an unmatched adaptability. As languages evolve, introducing new slang or adopting foreign terms, machine learning models can swiftly adapt, ensuring they remain current. This dynamism is crucial for businesses operating globally, ensuring they stay culturally relevant and linguistically accurate.
The Future of Linguistic Identity Detection
The trajectory of linguistic identity detection is on an upward curve, with technology propelling it forward at breakneck speed. Deep learning and neural networks, advanced facets of machine learning, are at the vanguard of this movement. Companies at the forefront, like Facebook's AI Research lab, are delving deep into these technologies to redefine our understanding of linguistic identities. As we look to the future, it's not just about identifying a language but understanding its context, history, and evolution. This deeper understanding can bridge cultural divides, fostering global collaboration and understanding.
Emerging Trends
Neural networks and deep learning carve out the next frontier in linguistic identity detection. Beyond mere identification, the focus is shifting towards understanding the intricacies of human communication. Sentiment analysis, for example, is gaining traction. Companies are keen on what language is being spoken and the sentiment and emotion behind the words. This holistic approach, championed by companies like Amazon and Microsoft, promises a future where systems can gauge linguistic origin and the essence of human expression.
Conclusion and Takeaways
The evolution from statistical models to machine learning in linguistic identity detection marks a significant leap in linguistics and technology. These models laid the foundation, but the digital age demanded more. Machine learning, championed by giants like Google and IBM, has risen to the challenge, offering unparalleled adaptability and precision. This shift benefits businesses, cultures, and societies, enabling tailored interactions and preserving linguistic nuances. With deep learning and neural networks spearheaded by companies like Facebook's AI Research lab and Amazon, the horizon expands further. We're moving towards detecting language and deeply understanding its emotion and context. This fusion promises a future where understanding transcends barriers, fostering universal communication and connection.
Get a glimpse into the future of business communication with digital natives.
Get the FREE report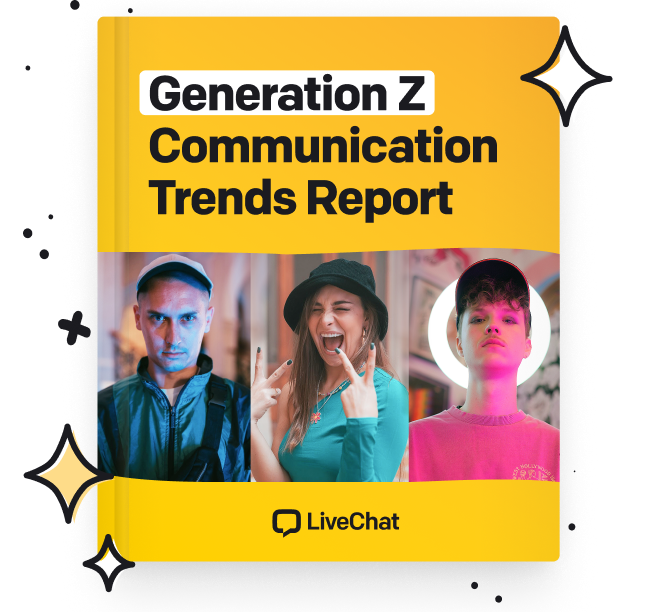