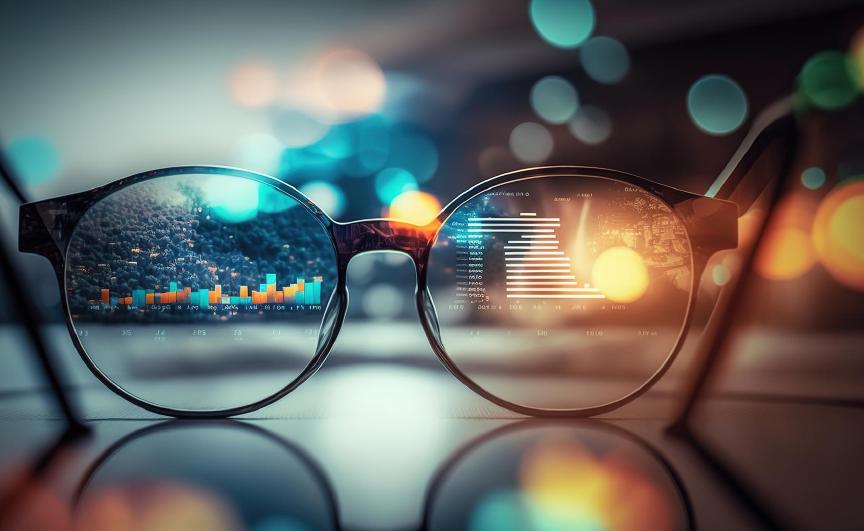
The text analytics industry is experiencing explosive growth, fueled by the ever-increasing volume of textual data generated and the growing need to extract valuable insights. This report digs into the current market size and analyzes historical data and industry trends. Keep reading to get all the details for the text analytics industry in 2024.
Current market size
According to research and industry reports by Mordor Intelligence, the global outlook of the text analytics market is valued at USD 10.49 billion in 2024 and is expected to reach USD 56.24 billion by 2029, growing at a staggering CAGR of 39.90%. Similarly, Market Research Future estimates the market to reach USD 11.91 billion by 2032, reflecting a consistent upward trajectory.
Growth drivers and trends
Several factors are propelling the market forward:
-
Surging data volumes: The exponential growth of social media, customer reviews, emails, and other textual data creates a vast pool for analysis.
-
Advancements in AI and NLP: Natural language processing (NLP) techniques like sentiment analysis, topic modeling, and entity recognition are becoming more sophisticated, unlocking deeper insights.
-
Growing demand for actionable insights: Businesses across industries seek to leverage text analytics for customer feedback analysis, market research, risk management, and more.
-
Cloud-based solutions: The rise of cloud-based text analytics platforms makes the technology more accessible and scalable for businesses of all sizes.
Regional breakdowns
The top market trends and growth exhibit regional variations:
-
The largest market share is North America due to early adoption and high IT spending.
-
Asia Pacific is estimated to grow fastest due to a sizeable tech-savvy population and government initiatives.
-
Europe has a mature market with solid regulations driving data privacy and security concerns.
-
Latin America, the Middle East, and Africa are in the early stages of adoption but show promising growth potential.
Future outlook:
The text analytics market is expected to maintain its high growth trajectory, driven by continuous advancements in AI, NLP, and the ever-growing need for data-driven decision-making. Businesses across industries will increasingly adopt text analytics solutions to gain deeper customer understanding, improve operational efficiency, and drive innovation in emerging markets.
Industry players and market share
The text analytics industry is dynamic, with diverse players vying for market dominance. Analyzing key players, their financial reports, market share statistics, and strategic moves provide valuable insights into the competitive landscape.
Major companies
Here's a profile of some prominent players:
-
IBM: Offers Watson Natural Language Understanding, a comprehensive NLP platform, and Watson Knowledge Catalog for data organization.
-
SAP: Provides SAP HANA Cloud for data management and SAP Customer Experience for text analysis in customer interactions.
-
Microsoft: Offers Azure Text Analytics to deliver sentiment analysis, topic modeling, and entity recognition functionalities.
-
Clarabridge: Specializes in customer experience analytics with sentiment analysis, topic identification, and voice of customer solutions.
-
SAS Institute: Offers SAS Text Analytics for text mining, sentiment analysis, and entity extraction.
-
Google Cloud: Employs Cloud Natural Language API for entity recognition, sentiment analysis, and text classification.
-
Amazon Web Services: Provides Amazon Comprehend for sentiment analysis, topic modeling, and entity recognition within the AWS ecosystem.
-
Genpact: Focuses on customer service and operational improvement using advanced text analytics capabilities.
-
Aylien: Offers a range of APIs for sentiment analysis, entity recognition, and topic modeling with multilingual support.
Market share analysis
Individual market share figures can vary based on methodology and chosen segments. Industry and market research reports may indicate the following:
-
IBM, Microsoft, and SAS hold significant market share. IBM is often ranked as a leader due to its extensive NLP solutions and Watson platform.
-
Cloud-based vendors like Google Cloud and AWS are gaining traction, driven by their scalability and integration with broader cloud ecosystems.
-
Specialist players like Clarabridge and Aylien carve out niches by focusing on specific industries or functionalities.
Mergers, acquisitions, and partnerships:
The industry has witnessed significant consolidation and collaboration:
-
IBM acquired Vivisimo in 2012, bolstering its search and text mining capabilities.
-
SAP bought KXEN in 2013, adding advanced text analytics to its portfolio.
-
Microsoft acquired Fast Search & Transfer in 2008, laying the foundation for Azure Text Analytics.
-
Genpact partnered with Google Cloud in 2022, accelerating their shared clients' cloud, data, and analytics modernization journeys.
These initiatives highlight the growing strategic importance of text analytics, financial data, and the drive for industry performance and comprehensive solutions.
The latest technological advances in text analytics
Let's look at some of the most significant developments shaping the text analytics landscape in 2024:
NLP on steroids
-
Transformer-based architectures: Models like LaMDA and Megatron-Turing NLG continue to break ground by processing language more contextually and generating human-quality text. This empowers applications like chatbots, dialogue systems, and machine translation to deliver more natural and engaging interactions.
-
Multilingual capabilities: NLP models are shedding their monolingual limitations and embracing multilingual proficiency. This allows for analyzing text across diverse languages, facilitating global information access and cross-cultural communication.
-
Personalized language models: Advances in fine-tuning techniques enable NLP models to adapt to specific domains and user preferences. This personalization unlocks industry-specific insights and tailors text analysis to individual needs.
Machine learning models evolve
-
Explainable AI (XAI) in text analysis: Demystifying complex models' "black box" nature is crucial for trust and interpretability. XAI techniques are integrated into text analysis, providing insights into how models arrive at their conclusions and enhancing decision-making transparency.
-
Federated learning for privacy-preserving analysis: Sensitive data often resides in silos, hindering collaborative analysis. Federated learning enables training models on decentralized datasets without data exchange, safeguarding privacy while unlocking shared insights.
-
Reinforcement learning for dynamic text: Models learn to interact with text in real time, adapting their responses based on feedback in dynamic environments. This paves the way for more interactive and engaging applications like personalized learning systems and adaptive dialogue platforms.
Sentiment analysis is super
-
Understanding emotions and nuances: Sentiment analysis moves beyond basic positive/negative classifications. Models now recognize complex emotions like sarcasm, humor, and cultural context, leading to more accurate and insightful sentiment understanding.
-
Identifying speaker intentions and motivations: Beyond just sentiment, models are beginning to grasp the underlying reasons behind language choices. This enables sentiment analysis to delve deeper into speaker personas, motivations, and potential actions.
-
Real-time sentiment analysis for agile decision-making: Sentiment analysis is becoming increasingly real-time, allowing businesses to monitor brand perception, customer feedback, and social media trends instantly, informing agile and data-driven decision-making.
Performance metrics of text analysis tools
The success of a text analysis tool hinges on its ability to accurately and effectively process and extract meaning from textual data. Various performance metrics come into play to assess this ability, each offering a unique perspective on the tool's strengths and weaknesses.
Here, we'll look at key metrics like accuracy, precision, and recall, exploring the key trends and their nuances and comparing different tools and approaches across diverse contexts.
Accuracy
Accuracy, the most intuitive metric, represents the overall proportion of correct predictions made from comprehensive data used by the tool. However, its simplicity can be misleading, especially in imbalanced datasets where one class dominates. For instance, a tool classifying all emails as "spam" might achieve high accuracy but fail to identify critical non-spam emails.
Precision and recall
Precision, measured as the ratio of correctly identified positives to the total predicted positives, indicates how precise the tool is in its classifications. Recall, on the other hand, gauges the proportion of actual positives that the tool correctly identifies.
Imagine a tool classifying customer reviews as positive, negative, or neutral. High precision ensures most "positive" classifications are genuinely positive. In contrast, high recall guarantees that most positive reviews are categorized as such. The ideal scenario involves balancing both metrics.
Comparing approaches
The performance of text analysis tools varies depending on numerous factors:
-
Task: Different tasks, like sentiment analysis or topic modeling, demand different metrics for meaningful evaluation.
-
Dataset: The training and testing data's size, quality, and complexity significantly impact performance.
-
Algorithm: Different NLP algorithms (like rule-based vs. machine learning) have inherent strengths and weaknesses.
Direct comparisons, therefore, should be made cautiously, considering the specific context and metrics employed. Here's an illustrative example:
The regulatory environment surrounding text analytics
The immense value extracted from text analysis sits amidst a complex web of regulations designed to protect data privacy and ensure the responsible use of technology. Understanding this regulatory landscape is crucial for organizations leveraging text analytics, as navigating compliance pitfalls can mean the difference between innovation and costly repercussions.
Data privacy regulations
-
General Data Protection Regulation (GDPR): Europe's GDPR sets a high bar for data privacy, requiring transparency, consent, and security measures throughout the text analysis process. Understanding data flows, anonymization techniques, and user consent mechanisms becomes paramount.
-
California Consumer Privacy Act (CCPA): California's CCPA empowers consumers with access, deletion, and opt-out rights regarding their data used in text analysis. Businesses must develop mechanisms to address these rights seamlessly.
-
Similar regulations: Privacy laws like Brazil's LGPD and China's PIPL are emerging globally, requiring organizations to adapt their text analytics practices to comply with local regulations in each jurisdiction they operate.
Industry-specific compliance
-
Healthcare: HIPAA in the US mandates strict data security and privacy controls for health information, influencing how healthcare organizations utilize text analysis for medical records analysis or patient feedback.
-
Finance: Financial regulations like the Gramm-Leach-Bliley Act (GLBA) in the US govern the use of customer data in the financial sector, impacting how banks and financial institutions can utilize text analysis for risk assessment or marketing campaigns.
-
Other industries: Depending on the industry, specific data security regulations, consumer protection, and intellectual property rights might apply to text analytics practices, requiring tailored compliance approaches.
Recent developments
-
Artificial intelligence regulations: Global discussions and proposals for ethical AI frameworks, including algorithmic bias and explainability, could impact the development and deployment of text analytics models, requiring transparent and fair algorithms.
-
Data localization requirements: Some countries are mandating data storage and processing within their borders, creating challenges for multinational organizations using cloud-based text analytics solutions.
Opportunities in the face of challenges
Navigating the regulatory landscape can seem daunting, but it also presents opportunities:
-
Building trust and transparency: Implementing robust data privacy practices can enhance customer trust and brand reputation, fostering long-term business value.
-
Driving innovation: Compliance can spur the development of secure and privacy-preserving text analytics solutions, fostering responsible innovation in the field.
-
Collaboration and standardization: Industrywide cooperation and the development of standardized approaches to compliance can ease the burden on organizations and accelerate the broader adoption of text analytics.
Industry insights
Text analytics is rapidly transforming various industries by extracting valuable insights from the ever-growing volume of textual data. Let's explore how this technology is revolutionizing the industry research four key sectors:
Finance
-
Fraud detection and risk management: Analyzing emails, transaction logs, and social media data helps identify suspicious activity and prevent real-time fraud.
-
Market sentiment analysis: Monitoring news articles, social media, and analyst reports allows investors to gauge market sentiment and make informed investment decisions.
-
Credit scoring and loan approval: Text analysis of financial documents and social media profiles enables more accurate credit assessments and personalized loan offers.
Challenges: Data security and privacy regulations, model explainability for fair lending practices.
Successes: Reducing fraud losses, identifying profitable investment opportunities, and improving loan approval efficiency.
Adoption rate: High and growing, driven by regulatory pressure and potential cost savings.
Healthcare
-
Clinical decision support: Analyzing medical records and patient notes helps doctors identify diseases, suggest treatment options, and improve patient outcomes.
-
Drug discovery and development: Analyzing scientific publications and social media data accelerates the drug discovery process and identifies potential adverse drug reactions.
-
Patient engagement and feedback analysis: Monitoring online reviews and surveys helps understand patient experiences and improve healthcare delivery.
Challenges: Ensuring patient data privacy, integrating text analysis with existing healthcare systems, and managing model bias and fairness.
Successes: Improving diagnosis accuracy, developing new drugs faster, and enhancing patient satisfaction.
Adoption rate: Moderate and growing due to privacy concerns and integration challenges.
Ecommerce
-
Product recommendation and personalization: Analyzing customer reviews and purchase history enables personalized product recommendations and targeted marketing campaigns.
-
Sentiment analysis of customer reviews: Understanding customer sentiment towards products and services helps improve product quality and customer satisfaction.
-
Chatbots and virtual assistants: Text analysis powers chatbots that answer customer questions, resolve issues, and personalize shopping experiences.
Challenges: Dealing with unstructured data, managing large datasets, and ensuring the fairness and explainability of recommendations.
Successes: Boosting sales conversions, improving customer satisfaction, and reducing customer service costs.
Adoption rate: High and rapidly growing, driven by the increasing importance of online shopping across consumer industries.
Customer Service:
-
Analyzing customer support tickets and social media mentions: Identifying common issues, understanding customer sentiment, and improving customer service resolution time.
-
Personalizing customer interactions: Using chatbots and virtual assistants powered by text analysis to provide immediate and personalized support.
-
Predicting customer churn: Identifying customers at risk of leaving and proactively engaging them to improve retention.
Challenges: Training chatbots to understand natural language nuances, ensuring data privacy, and integrating text analysis with existing CRM systems.
Successes: Reducing customer churn, improving customer satisfaction, and optimizing resource allocation in customer service departments.
Adoption rate: Moderate and growing, with larger companies leading the way.
Conclusion
This comprehensive report has shown the exciting world of text analytics, analyzing its current market size, exploring growth drivers and trends, and examining its transformative impact across various industries. It also looked at the latest technological advancements, key performance metrics, industry statistics, and the evolving regulatory landscape surrounding this powerful technology.
Get a glimpse into the future of business communication with digital natives.
Get the FREE report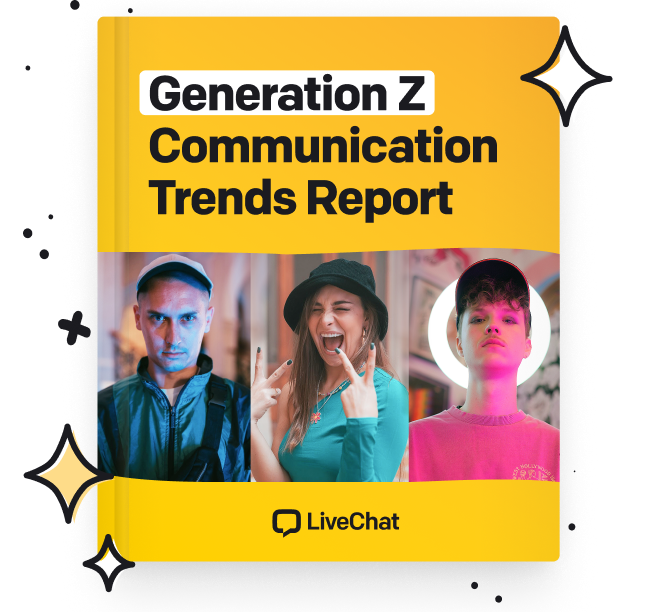